Early Lung Cancer Prediction approach based on Gene Disorder using Improved GA and Decision Tree approach
Abstract
Abstract:
This research supports changes and variation of DNA sequence based on mutation of DNA gene arrangement over a gross chromosome irregularity. This change in gene disorder leads to new infectious diseases or abnormal changes in the human cellular body. This paper discusses the prediction of lung cancer traces, primarily due to mutations due to clinical and environmental factors exposure. The proposed model predicts the genetic phenotype from observed patients' relevant gene factors and non-genetic traces of lung cancer. Results and analysis show that the prediction rate supports an average of 73.81% of gene disorders compared with ACO and GA approaches.
Background:
The survey shows that most genetic diseases are the immediate consequence of a mutation in multiple genes. A survey and analysis of research work that supports changes and DNA variation of gene sequence, based on mutation DNA gene arrangement to a gross chromosome irregularity.
Objective:
This research aims to predict lung cancer cells based on genetic phenotype from its relevant gene factors and non-genetic traces of lung cancer from observed patient datasets.
Methods:
Major changes in gene disorder lead to abnormal changes in the human cellular body and hence the growth of cancerous tissues. The paper discusses the prediction of lung cancer traces, primarily due to gene mutations and exposure to climatic and environmental factors. An improved GA and decision tree approach as a classifier is designed and developed to support early prediction.
Results:
Analysis shows that the prediction accuracy rate supports an average of 73.81% of lung cancer based on gene disorder compared to the ACO and GA approaches.
Conclusion:
The result of the experiment shows that the approaches give more accuracy than the previous approaches.
1. INTRODUCTION
Today's research [1] on the healthcare sector generates much patient medical data. The health information gathered corresponds to disease identification [2], which requires the treatment type and diagnosis methods that may differ from an individual. Most genetic diseases are the immediate consequence of a mutation in one gene. In any case, a standout amongst the most troublesome issues ahead is to promote and illustrate how genes add to diseases that have a perplexing example of legacy, for example, in the instances of diabetes, asthma, cancer, and emotional sickness. Historical analysis [3, 4] suggests that, in any case, no gene has the energy to determine whether a human will build up the disease or not. More than one mutation is likely required before the disease is predicted, and various genes may make an inconspicuous commitment to a human being's powerlessness to lancer disease. Gene modification may influence how a human responds to ecological components. Primarily, gene disorder is based on signal-based search and gene structure sequence similarity search (aka gene analysis).
1.1. Problem Definition
Analysis suggests that determining lung cancer at an early stage is a major challenge due to the complexity of patient data available in absolute format. Due to climatic and food types, patients’ demographical data is observed by society. Hence, their clinical and pathological data help as molecular details behind the prediction of lung cancer gene alterations in the context of prediction at an early stage. A genetic disorder [2] can lead to serious implications or disease, which could be brought on by a variation from the norm in an individual's genome or an individual's entire change in genetic arrangement. Hence detecting an appropriate gene is a challenge [5]. Another primary concern is on type of analysis carried out. Most earlier research work [6] conducted in this domain prevails primarily on patient CT, MRI or XRay images.
1.2. Knowledge Gap
The variation of DNA sequence [7] is based on mutation. It can be extended from infinitesimal to major changes as a discrete mutation in the solitary base of DNA gene arrangement to a gross chromosome irregularity, including the expansion or subtraction of a whole chromosome or set of chromosomes. Some genetic disorders are acquired from straight parents or fathers [4, 8], while other genetic diseases are brought about by gaining changes or mutations in a previous gene or gathering of genes. Gene mutations can happen haphazardly or based on new infectious diseases or abnormal changes in the human cellular body [9].
As the literature survey on lung cancer prediction and analysis suggests, earlier approaches to designing and implementing lung cancer tumor cells from noisy images dataset and using computational models such as SVM [7] and regression approaches [10] yield no proper optimal results. The vital objective of this research paper focuses on applying optimization approaches with data analytics to focus on the prediction of lung cancer based on variable changes in DNA structures from different patients [3] as a data set in a human-sensible structure.
The role of data analytics supports the entire technique of applying appropriate computational models [11], including new strategies for the learning revolution based on appropriate genetic datasets. The primary objective of this research paper is two-fold (a) to design an improved genetic disorder prediction approach for (Mendelian) monogenetic inheritance, mono gene and multi-gene changes (b) to support an early understanding of the basic ambiguities of genetic disorders and aspects of lung cancer disease. A cohort approach of community practice among patients is discussed in section 3.0 to analyze and evaluate the clinical decision-making for predicting lung cancer among demographical society. The clinical complexity of the patient is analyzed in detail, and the process of lung cancer-based observed symptoms for a decision‐making approach can arrive as an outcome of the proposed work.
The paper is summarized in Section 1, which introduces the challenges in the gene-disorder approach and the need for research in this domain. Detailed literature review and analysis are carried out on the decision tree and other computational models in Section 2. Section 3 discusses the IDGPA model using the improved decision tree model and how genetic disorders can be predicted. Section 4 shows the experimental test dataset adopted for this work and the analysis. The results and performance of IDGPA compared with other approaches are also discussed in section 5. Elaborates on the limitations, and Section 6 summarizes the work and concludes with the need for future work.
2. MATERIALS AND METHODS
2.1. Related work and Research Gaps
Survey [5, 12] suggests that consistent updates on diagnosing and treating lung cancer from the clinical dataset are increasingly necessary for prediction accuracy. The need for a predictive analytical model for health knowledge and providing scientific decision-making for immediate health care is demanded from health caretakers and experts [13]. Most gene disorder discovery approaches employ fast computational gene discovery analysis [14] models, which incorporate Bayesian classification, random forest, neural systems, and backpropagation approaches [2] such as gene-alteration (taking into account neural systems), the k-closest neighbor classifier (adaptation by similarity), and gene mutation model [15].
Classification models play a major role in the adaptive grouping of genes based on the partition of gene data. Computational approaches apply various fast classifiers tags for the gene for analysis. Data structural models and algorithms such as decision trees [10, 16] and random forests [4] are well-known top-down ways of gene grouping/classifiers into leaf and node divisions until the whole set has been examined. Neural network models are nonlinear prescient devices that gain from an arranged data set and are then connected to new, bigger sets. Genetic calculations are similar to neural systems, consolidating characteristic determination and transformation. The closest neighbor uses a prepared set of data to gauge the likeness of a gathering and handles data utilization such that the resultant data can be used to dissect the test data. In genetic studies, these strategies have uncovered intriguing discoveries, particularly in the heritable inclination to contract particular sicknesses, Decision Trees play the real part, and this is a choice bolster device that contains a tree-like diagram of choices and conceivable outcomes.
In order to illustrate the general system that is received in the utilization of choice tree calculations and the investigation of DNA data sets associated with instances of genetic issues, they have typically been used as a part of various real-world situations ranging from operations exploration to ordering specie. Inbamalar and Sivakumar proposed a method [12] for cancer prediction using the genomic signal processing method. This approach is based on DNA sequence analysis for cancer prediction. The proposed algorithm is found to improve cancer prediction models, and its performance is compared with existing methods.
A novel approach to predict CpG islands is discussed in [5], where a detailed analysis of DNA sequences using discrete wavelet transforms is elaborated. However, the primary objective focuses on increasing the sensitivity to the maximum and reducing noise. This approach is well suitable for the prediction of tumor suppressor genes.
Prediction of lung cancer using genomics profiling and mutational analysis helps in recent research work [15, 17] and is well accepted by clinical experts and the medical research community [14]. Numerous review works [18, 19] on genomic alteration identification approaches in lung cancer have a major impact on therapeutics [20] as well as when traces represent alterations of “oncogenic drivers.” Inbamalar et al. [7] devised a research work [11] that applies methods to identify protein-coding regions over DNA sequences based on wavelet transformation of DNA sequences. This paper's main aim supports increasing prediction accuracy and reducing the noise as much as possible using Coif let approach [21].
3. IDGPA FRAMEWORK
This work is proposed for lung cancer detection based on gene disorder based on the decision tree C-4.5 [19] and genetic algorithmic approach. The Framework of the proposed model IDGPA is shown in Fig. (1). Decision Tree C-4.5 is preferred in this approach since the generated rules demand higher accuracy and low algorithmic complexity.
Analysis and survey show that in lung adenocarcinoma, gene-types TP53 are found to be a frequently mutated gene based on somatic mutations, which is close to 73% of patient samples. The decision tree C-4.5 algorithm trains a data set based on which inference rules can be generated. The inference rules [6] are optimized for the rules based on the genetic algorithm. The decision Tree is generated, as shown in Fig. (2), based on patient medical and environmental data analysis.
The decision tree generated has 9 leaves; the tree size is 9, and the following decision rules can be created. The decision tree defined above identifies that if the patient is a smoker or has any other health-related issues, including health symptoms like wheezing and chest pain, the patient has a high chance of having cancer due to a genetic disorder. Also, a non-smoker who is coughing has very few chances of getting affected by lung cancer than a smoker. After generating the decision tree and discovering the relationships among various attributes from the training data set, it is necessary to obtain the prediction accuracy of the decision trees [22] (Table 1).
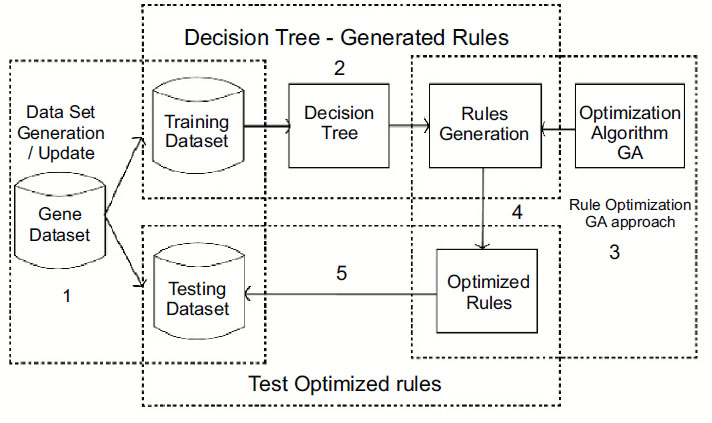
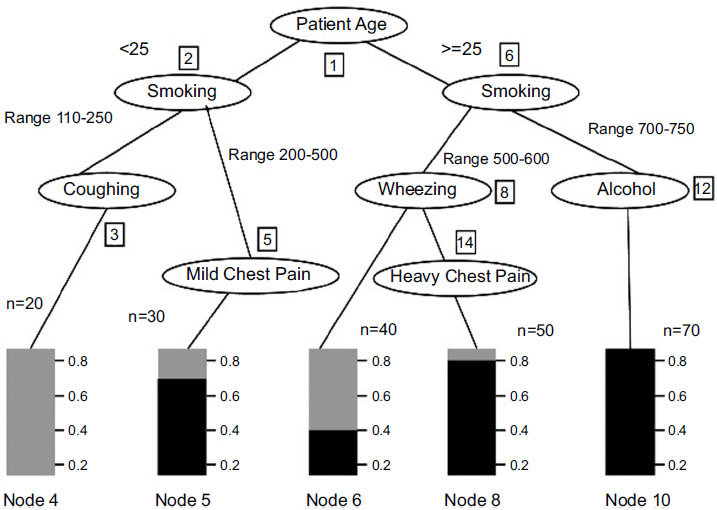
Prediction | < 60% | >60% |
---|---|---|
<60% | 71.78 | 28.20 |
>60% | 27.38 | 67.25 |
Accuracy | 29.25 | 71.50 |
Decision rules are applied to the test data set for this process, and a respective confusion matrix has been obtained.
Possibility_GeneCancer > 90%:
If Patient_Smoking = 1 & Observed_Wheezing =1 & Pain_Chest =1
Possibility_GeneCancer > 70%:
If Smoking = 1 & Wheezing =1 & Chest.Pain = 0 & Cough =1
If Smoking = 1 & Coughing =0 & Chest.Pain =1
Possibility of Genetic Cancer > 60%
If Smoking = 1 & Wheezing =1 & HeavyChestPain = 0 & Coughing =0
If Smoking = 1 & Wheezing =0 & Coughing =1 & MildChestPain =1
If Smoking = 1 & Wheezing =0 & Coughing =1 & MildChestPain =0
Possibility of Genetic_Cancer > 60%
If Smoking = 1 & Wheezing =0 & Coughing =0
If Smoking = 1 & Coughing =0
If Smoking = 1 & Coughing =0 & HeavyChestPain =0.
By applying the weight-based decision tree, the tree structure is based on the cell range as the root node and the obtained rules are as follows:
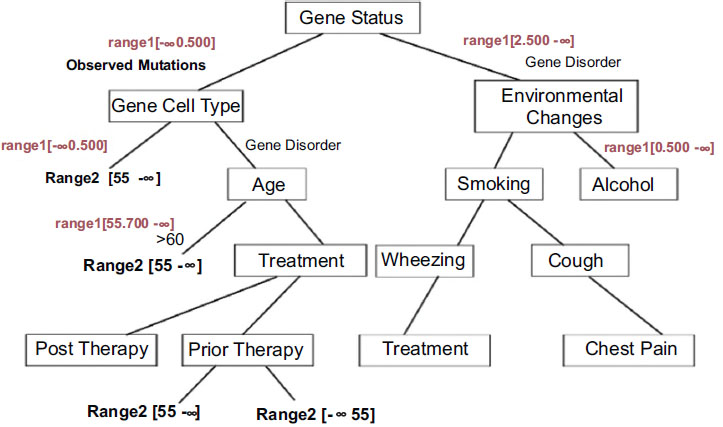
The decision tree generated with appropriate conditions based on gene therapy is shown in Fig. (3). Analysis shows that gene disorder with EGFR mutations is a strong predictive factor for improved outcomes with gefitinib therapy. The analysis also suggests that patients with no identifiable EGFR mutations may possess clinical parameters and may have a higher probability of having mutations, leading to a worse outcome of lung cancer ACD based on gefitinib therapy.
The decision tree T can be generated from the set of patient medical samples P. Attributes can be labelled as 'a' value, the number of classes Ni (i=1, 2,...n), and the set Pi can be defined as samples belonging to class Ni.
![]() |
(1) |
Here si ϵ Pi is a set of related features selected for analysis. Set P can be divided into values representing the major features of gene-disorder analysis and lung cancer. Si = Pi/p, which defines the matching probability of prediction. Set Pi can be subdivided into sub-sets of data values ‘m’.
![]() |
(2) |
Here I is the information feature obtained based on patient data as defined in Eqn (1)
Information gain,
![]() |
(3) |
The generated rules are based on the GA algorithm, which considers chromosomes with multiple genes. Datasets include attributes, which the GA approach considers as chromosomes. IDGPA approach divides chromosomes into genes, which correspond to attributes. Each individual chromosome represents a classification rule (Fig. 4).
GA analysis is carried out on multiple datasets, representing a classification rule for chromosomes. Chromosomes generate all possible problem solutions and new adaptive rules based on changing features selected from the dataset and achieve consistent accuracy.
The whole chromosome can represent a completed rule IF-THEN-ELSE. Genes specify the left-hand side of the classification rules constructed by genes, which correspond to the characteristic attributes of characteristic genes. The right side of the classification rule is constructed by genes that correspond to the class attribute as class genes. The final rule set will be sorted by the quality of the rule. Rule set realized on the new sample dataset; the first rule is defined as the best rule. The rule is considered the best rule only if it recognizes the sample; the next rule can be selected. Gene chromosomes, defined as rules, will compete for the population's priority. During gene evolution, consideration is provided for characteristic genes that can only participate in evolution, while class genes cannot participate in the process.
Chromosomes can be defined as a fixed length and possess gene parts such as weights, operators, values, and gain ratios. GA analysis in Table 2 shows that the prediction accuracy of lung cancer is 71.5%, and for the prognosis of lung cancer, it is 68.5% [23].
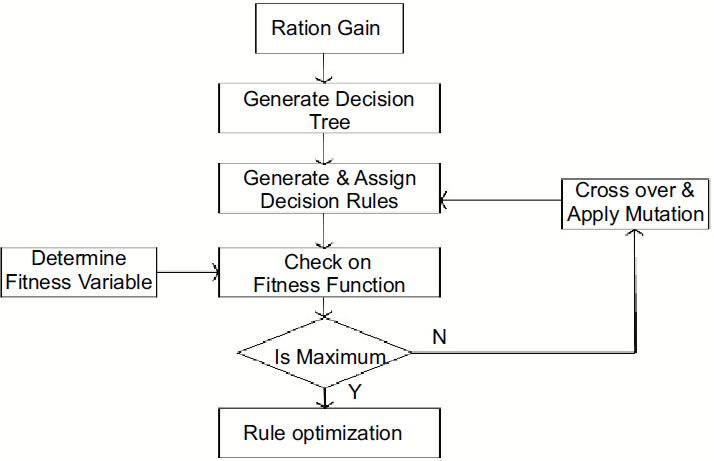
Prediction | Disease Free | Progressed | Recurred |
---|---|---|---|
Disease Free | 89 | 2 | 7 |
Progressed | 14 | 39 | 0 |
Recurred | 28 | 2 | 19 |
Accuracy | 68.5 |
Ref. No. | Age | G | Smoking Status | Stage | KIF5B-MET Variant | Histology | Treatment | OS Mon | Dead/ Alive |
---|---|---|---|---|---|---|---|---|---|
1. | 21 | M | No | III | M14; K24 | ADC | Supportive | > 15 | Alive |
2. | 49 | F | No | IV | M13; K24 | ADC | (PD after 26 mon) Bvz/Pem-cis and Pem SAIT301 (PD) crizotinib (10 mon), then other salvage therapies |
>32 | Dead |
3 | 36 | F | Yes | IV | M15; K24 | ADC | Crizotinib (PR) | >10 | Alive |
4 | 72 | F | No | IV | M15;K24 | ADC + PSC |
Pem-Cis (PD) | 4 | Dead |
5 | 78 | M | Yes | IV | M15; K24 | PSC | Supportive | 2 | Alive |
4. RESULTS AND DISCUSSION
This section discusses the performance analysis of IDGPA, whose results primarily depend on the lung cancer disease prediction time taken based on the age and smoking of candidates interviewed obtained from the dataset. The analysis uses five different datasets classified as DA1, DA2, DA3, DA4, and DA5. The datasets collected for various parameters involved in lung cancer predictive attributes involve a patient’s lifestyle, medical diagnosis, and gene details which may support the prediction of small lung cancer at an early stage. Figs. (5 and 6) show the performance of IDGPA over GA, which converges to local optima since the decision tree adopts convergence based on the hypothesis achieved towards the prediction of small lung cancer based on medical metrics and behavioural aspects (Table 3).
This research uses 260 diagnostic lung cancer ADC and 28 pulmonary sarcomatoid carcinoma patient specimens identified as negative for EGFR, KRAS, or RET mutations.
Data collected from the interviewing members including genetic behaviour, attitude and patient history, demographic characteristics of the region, occupation, information about
specific exposures at work or from hobbies, medical history, and family history of gene characteristics among a close relative are shown in
The environmental prediction metrics involve analytical air pollution, alcohol use, dust allergy, occupational hazards, genetic risk, chronic lung disease, balanced diet, obesity, smoking, passive smokers, chest pain, coughing of blood, fatigue, weight loss, breath shortness, wheezing, swallowing difficulty, clubbing of fingernails, frequent cold, dry cough, snoring level during sleep. Any individual who has never smoked or has smoked less than 100 cigarettes in their lifetime is always a non-smoker.
A smoker is always an individual who smokes a minimum of 10 cigarettes per day or at least 100 cigarettes in a year. A former smoker can be considered an individual who has smoked at least 100 cigarettes in their lifetime but quit smoking more than 12 months before lung cancer diagnosis (for case patients) or before the interview.
Fig. (5) explains the role of lung cancer metrics observed for the dataset under analysis compared to IDGPA and GA schemes.
Any genetic disorder incorporates major changes in the medical analysis due to which health immunity may be affected. Four different datasets were used for analysis, based on age as a major parameter which metric IDGPA shows minimal time to predict GA. Fig. (6) shows IDGPA performance over GA in terms of the smoking behaviour of patients. IDGPA suggests a higher prediction rate with minimal time IDGPA shows its performance in terms of accuracy of prediction rate and the time taken to predict as part of this research work.
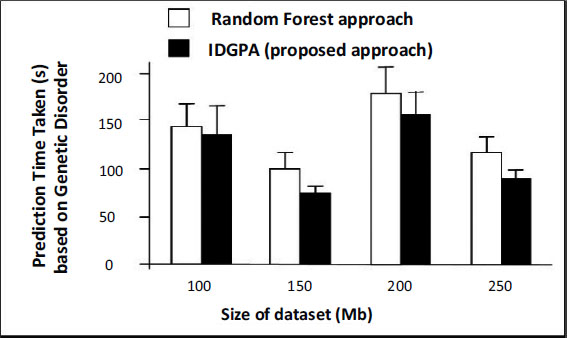
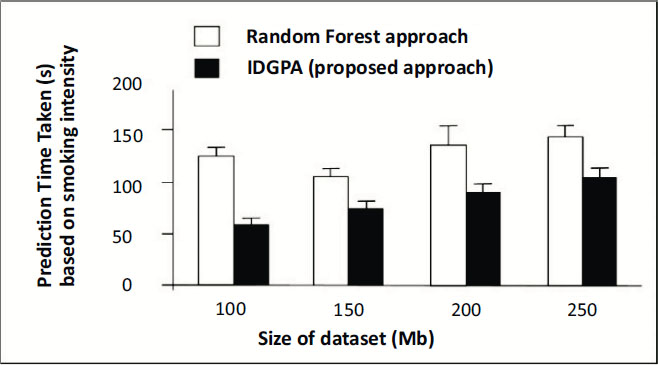
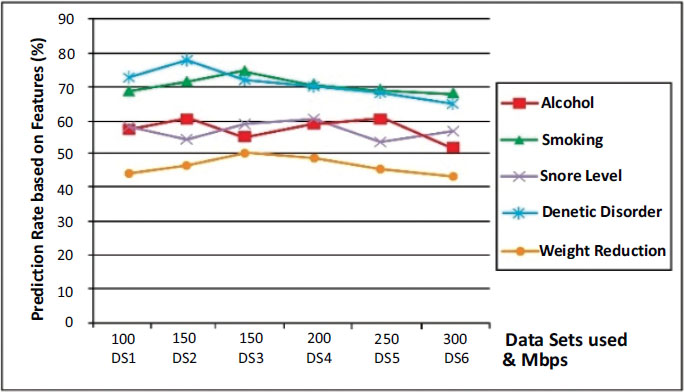
A comparison of major influential attributes which lead to lung cancer is shown in Fig. (7) over the IDGPA approach. Genetic Disorder shows higher prediction criterium as analysis over other attributes, while weight reduction does not have a major impact on cancer prediction as similar to other attributes. It could also be understood from Figs. (6 and 7) that IDGPA shows an optimal measure to perform among large datasets.
Fig. (8) shows that IDGPA supports an average of 87.27% of the prediction rate, while ACO is better than GA, but it performs linearly for differing datasets such that its average lies at 65.88% while GA shows an average of 73.81%.
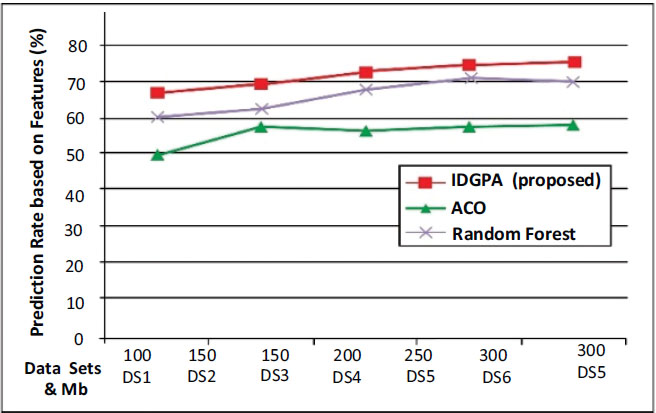
5. LIMITATIONS AND FUTURE WORK
IDGPA proposed in this research suggests a better prediction rate using the decision tree approach using GA, but it also has the following limitations.
(a). Due to the large data size, IDGPA requires more time taken to converge to global optima, although GA supports local optima for the recurrence of observed sets
(b). The observed time taken for the prediction of gene disorder is moderately higher for IDGPA compared to other ML approaches.
CONCLUSION
Studies and analysis show that lung cancer-based gene changes are found to be modified during a person's lifetime instead of being inherited from more parents. Lung cancer is exhibited primarily due to mutations from exposure to environmental factors such as cancer-causing chemicals such as chewing tobacco or cigar smoke. IDGPA model predicts the genetic phenotype from its relevant gene factors and non-genetic (covariates, e.g., environmental variables) as information measured on the patient individuals in the training set. The observed pattern of features that attribute values to lung cancer decision attributes per analysis is considered. IDGPA analysis shows that the prediction rate supports an average of 87.27% of gene-disorder compared with ACO and GA approaches. The traces of linear lung cancer datasets are considered for analysis, whereas for different datasets, the ACO average lies at 65.88%, while GA shows an average of 63.27%. Future work can be suggested toward the need for an adaptive prediction approach using optimization models.
ETHICS APPROVAL AND CONSENT TO PARTICIPATE
Not applicable.
HUMAN AND ANIMAL RIGHTS
Not applicable.
CONSENT FOR PUBLICATION
Not applicable.
AVAILABILITY OF DATA AND MATERIALS
Not applicable.
FUNDING
None
CONFLICT OF INTEREST
The authors declare no conflict of interest, financial or otherwise.
ACKNOWLEDGEMENTS
Declared none.