Initial Investigation of Athletes’ Electrocardiograms Acquired by Wearable Sensors during the Pre-exercise Phase
Abstract
Aim:
The aim of this study is to support large-scale prevention programs fighting sport-related sudden cardiac death by providing a set of electrocardiographic features representing a starting point in the development of normal reference values for the pre-exercise phase.
Background:
In people with underlying, often unknown, cardiovascular abnormalities, increased cardiovascular load during exercise can trigger sport-related sudden cardiac death. Prevention remains the only weapon to contrast sport-related sudden cardiac death. So far, no reference values have been proposed for electrocardiograms of athletes acquired with wearable sensors in the pre-exercise phase, consisting of the few minutes immediately before the beginning of the training session.
Objective:
To perform an initial investigation of athletes’ electrocardiograms acquired by wearable sensors during the pre-exercise phase.
Methods:
The analyzed electrocardiograms, acquired through BioHarness 3.0 by Zephyr, belong to 51 athletes (Sport Database and Cycling Database of the Cardiovascular Bioengineering Lab of the Università Politecnica delle Marche, Italy). Preliminary values consist of interquartile ranges of six electrocardiographic features which are heart rate, heart-rate variability, QRS duration, ST level, QT interval, and corrected QT interval.
Results:
For athletes 35 years old or younger, preliminary values were [72;91]bpm, [26;47]ms, [85;104]ms, [-0.08;0.08]mm, [326;364]ms and [378;422]ms, respectively. For athletes older than 35 years old, preliminary values were [71;94]bpm, [16;65]ms, [85;100]ms, [-0.11;0.07]mm, [330;368]ms and [394;414]ms, respectively.
Conclusion:
Availability of preliminary reference values could help identify those athletes who, due to electrocardiographic features out of normal ranges, are more likely to develop cardiac complications that may lead to sport-related sudden cardiac death.
1. INTRODUCTION
Regular physical activity has proven beneficial for cardiovascular health. However, in a few cases, increased cardiovascular load during exercise in people with an underlying, often unknown, cardiovascular abnormality can trigger sport-related sudden cardiac death (SrSCD). SrSCD is the leading medical cause of death across all sports [1-4]; it is an unexpected death occurring while performing a sports activity or within one hour from its cessation [5]. Sometimes, SrSCD may also occur after exercise at rest or during sleep [1]. When occurring in athletes under 35 years old is considered independent by other cardiovascular complications due to aging [4].
Prevention remains the only weapon to contrast SrSCD. Worldwide, the need to implement pre-participation screening for young individuals approaching sports [6] and to potentiate screenings for athletes already practicing sports [7] is widely debated with the main goal to balance among saved lives, economic costs, and legal, ethical, and psychological issues [5]. Pre-participation screening represents the first-line test for the diagnosis of heart disease in sport participants. Currently, it includes clinical and family history, physical examination, rest and stress 12-lead electrocardiographic test [8]. Instead, screening of athletes already practicing sports consists of specific tests [9] regularly but not too frequently (typically once a year) performed to identify cardiovascular abnormalities predisposing to SrSCD. Unfortunately, most screenings are usually recommended for competitive athletes, while amateur athletes and people occasionally practicing sport are typically left to optional, sometimes self-made, evaluations of their health status [7].
It is well known that athlete’s heart undergoes some physiological adaptations related to exercise. These changes may depend on age, sex, ethnicity, type of sport, intensity of training, amount of training (training session duration times weekly training rate), and number of practiced sports [8, 10, 11]. These physiological changes may reflect on the electrocardiogram (ECG) that results deviated with respect to normal in 10-12% of athletes [12-14]. Sometimes, these physiological deviations may be confounded with a cardiovascular pathology and lead to unreasonable disqualification from sport [8]. Other times, pathological ECG deviations in athletes are wrongly interpreted as physiological adaptations with dramatic outcomes [1]. Thus, specific athlete’s ECG interpretation guidelines are fundamental when screening athletes [12].
So far, international recommendations for athlete’s ECG interpretation have been developed only for resting standard 12-lead ECG for which normal reference values and pathological thresholds, often lead dependent, have been indicated [12]. However, the resting standard 12-lead ECG is a clinical test typically done in hospitals or healthcare facilities and thus occasionally (usually during pre-participation screening) performed. Differently, some wearable physical-activity monitoring sensors, commonly used to optimize exercise dose and intensity, are also able to reliably record the ECG and could provide useful clinical information on athlete’s health status at each training session, especially if combined with software applications for automatic ECG interpretation [7, 15-19]. Typically, wearable sensors provide a reduced number of ECG leads, which do not necessarily match a subset of the 12 standard ECG leads. Additionally, acquisition settings in which they are typically used do not match the strict protocols followed in the clinical setting. Consequently, known reference values normally used in clinics for resting ECG should not strictly apply, even if they may provide some insights into the athletes’ health.
As far as we know, no normative reference values have been proposed for ECG of athletes acquired with wearable sensors preceding the training session. The pre-exercise phase, consisting of the few minutes immediately before the beginning of the training session, does not correspond to resting (since the athletes do not lie and can freely move and talk) or to exercise (which has not started yet and does not occur in controlled conditions as in clinics, anyways). Yet, it may represent a good moment to frequently (ideally before each training session) evaluate athlete’s heath since movement artefacts, increased respiration, and noise are still sufficiently low to allow a reliable ECG analysis (differently from what typically occurs while practicing exercise). Availability of reference values for the pre-exercise ECG could help to identify and stop those athletes who, due to ECG features out of normal ranges, are more likely to develop cardiac complications during the subsequent training session.
The aim of this study is to support large-scale prevention programs fighting SrSCD by providing a set of ECG features representing a starting point in the development of normal reference values for the pre-exercise phase. Thus, the objective is to perform an initial investigation of athletes’ ECGs acquired by wearable sensors during the pre-exercise phase.
2. MATERIALS AND METHODS
2.1. Study Population
Experimental data for this study were taken from the freely available Sport Database [15], including 126 cardiorespiratory datasets from 81 athletes (53 males and 28 females), and from the Cycling Database of the Cardiovascular Bioengineering Lab of the Università Politecnica delle Marche (Ancona, Italy), including 12 cardiorespiratory datasets from 12 athletes (10 males and 2 females). Both databases contain ECG recordings (one lead ECG; sampling frequency 250 Hz) acquired in Caucasian athletes and recorded through the wearable chest strap BioHarness 3.0 by Zephyr (www.zephyranywhere.com). The wearable chest strap BioHarness 3.0, schematically represented in Fig. (1), is a physiological monitoring telemetry device that consists of a chest strap and an electronic module attached to the strap. The device detects and transmits single-lead ECG signals to be received by Bluetooth / USB qualified ECG instruments. The acquisition protocol was the same for the two databases; according to it, each acquisition included a 5-min long pre-exercise phase, a training phase of varying length, and a 5-min recovery phase [15]. The acquisition protocol was not always strictly respected by the athletes so that pre-exercise ECG is not available for all of them. Among all available athletes’ ECG recordings, only those acquired during the pre-exercise phase in healthy athletes (i.e., with no previous or current history of cardiorespiratory diseases and not taking any drugs) were considered suitable for the present study. In the case of multiple acquisitions for a single athlete, only one dataset was considered.
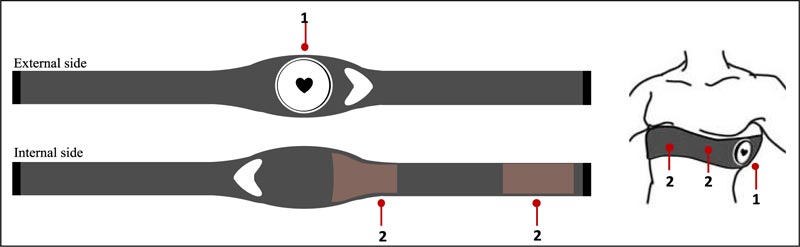
The acquisitions were freely performed on the field (not in hospitals or in clinics) from athletes, who voluntarily asked to participate in the study. All athletes gave their written consent prior to data acquisitions, which were performed in compliance with the ethical principles of the Helsinki Declaration and approved by the institutional expert committee.
2.2. Sport Classification
Sports practiced by athletes were classified according to Mitchell’s criterion [10]. Each sport class was defined on the basis of a different combination of dynamic and static components (Fig. 2). Specifically, three main classes, namely A, B, and C, were identified in relation to the dynamic component, which is measured in terms of the achieved maximal oxygen uptake (Max O2; %): less than 40% (A), between 40% and 70% (B) and over 70% (C). Each class was further divided into three subclasses, namely I, II, and III, in relation to the static component, which is measured as the reached maximal voluntary contraction of muscles (MVC; %): less than 20% (I), between 20% and 50% (II), and over 50% (III).
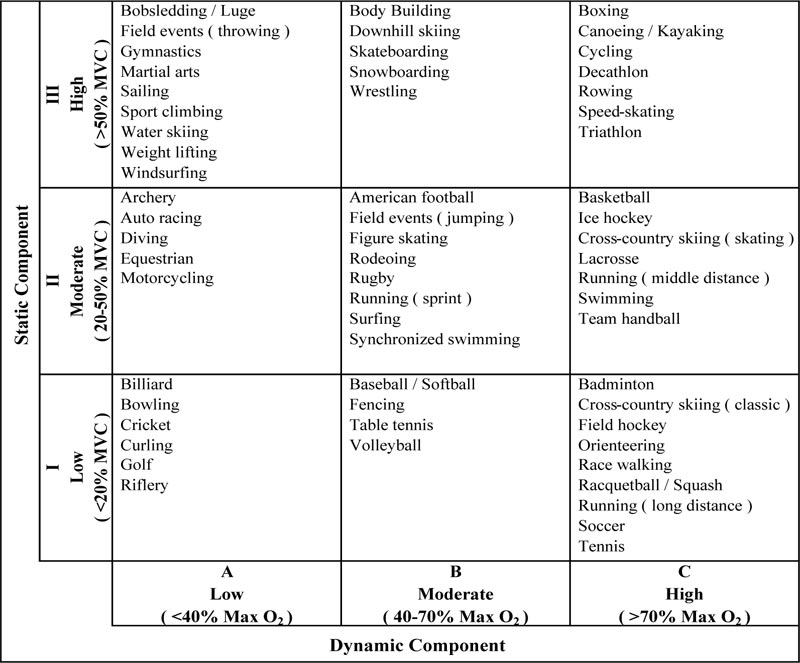
2.3. Experimental Electrocardiographic Data
The first clean 10 s ECG window (the same window length conventionally used in the standard 12-lead ECG) was extracted from the ECG of each athlete acquired during the pre-exercise phase. The 10 s ECG was bandpass filtered (cut-off frequencies: 0.5 Hz and 50 Hz) in order to remove baseline wander and high-frequency noise. R-peak positions were automatically identified [20] and used to compute mean RR interval (mRR; ms), heart rate (HR, computed as 60/(mRR∙10-3); bpm), and heart-rate variability (HRV, computed as the standard deviation of the RR intervals; ms). Successively, the median cardiac beat (mBeat) was computed and used as representative of the athlete’s 10 s pre-exercise from which the following landmarks have been automatically identified [21]: peak of the R wave (Rp), onset of the QRS complex (QRSon), offset of the QRS complex (QRSoff), peak of the T wave (Tp) and offset of the T wave (Toff). Correct location of the automatically identified landmarks was confirmed by visual inspection of an expert cardiologist. The landmarks were eventually used to evaluate the ECG features typically used in clinics to assess cardiovascular risk [12] in addition to HR and HRV, which are: QRS duration (QRS-duration, computed as QRSoff – QRSon; ms), ST level (ST-level, computed as [(mBeat(QRSoff) – mBeat(QRSon)] / 0.1; mm), QT interval (QT-interval, computed as Toff – QRSon; ms) and corrected QT interval (QTc-interval, computed as QT-interval / mRR1/2, according to the Bazett’s formula [22]; ms).
2.4. Statistics
Healthy athletes with available ECG recording during the pre-exercise phase were stratified according to age (≤35 years and >35 years) [4] and practiced sport [10]. Distributions of ECG features were reported in terms of 50th[25th;75th] percentiles, where the 25th and 75th percentiles represent the lower and upper bounds of the suggested range, respectively. Wilcoxon ranksum test was performed to assess statistical differences in ECG features distributions in subclasses and against reference values available in the literature [12, 23, 24] for standard 12-lead resting ECG. The statistical significance level was set at 0.05. The statistical analysis was performed using the Statistics and Machine Learning Toolbox in MATLAB R2018b.
3. RESULTS
Overall, 51 athletes (38 males and 13 females) were found to be eligible for the study. On average, the athletes were 29±11 years old and exercising 4±1 times a week. Practiced sports were basket, cycling, fitness, jogging, middle-distance running, tennis, and CrossFit. Consequently, all practiced sports belonged to class C. Specifically, 8 athletes were practicing sports in class CI (tennis and jogging), 15 in class CII (basket and middle-distance running), and 28 in class CIII (cycling, fitness, and CrossFit). Further information on the population of athletes involved in the study is reported in Table 1.
Table 2 contains preliminary values for athletes’ ECGs acquired by wearable sensors during the pre-exercise phase. When stratifying for age, for the athletes (N=39) 35 years old or younger, no statistically significant differences were observed between median values of any ECG feature distribution over different C subclasses. Instead, for athletes (N=12) who were older than 35 years, QTc-interval was significantly longer in CII than in CIII.
Several statistically significant differences were observed when comparing pre-exercise ECG feature distributions against 12-lead resting ECG (Table 2) [12, 23, 24]. In general, athletes showed a significant reduction of HRV and a significant QRS-duration prolongation. Additionally, the younger ones (≤35 years) tended to show a significant shortening of the QT-interval and QTc-interval.
Sport | N | Sex Male/Female |
Age (years) |
Weight (kg) |
Height (cm) |
Sport Class |
Weekly training rate |
---|---|---|---|---|---|---|---|
Basket | 6 | 6/0 (0) |
22 ± 4 (0) |
73 ± 9 (0) |
181 ± 5 (0) |
CII | 4 ± 0 (0) |
Cycling | 9 | 7/2 (0) |
35 ± 17 (0) |
64 ± 8 (0) |
172 ± 6 (0) |
CIII | 3 ± 1 (0) |
Fitness | 8 | 6/2 (0) |
25 ± 5 (0) |
71 ± 14 (0) |
173 ± 7 (0) |
CIII | 4 ± 1 (0) |
Jogging | 4 | 2/2 (0) |
24 ± 1 (0) |
63 ± 14 (2) |
172 ± 8 (2) |
CI | - (4) |
Middle-distance running | 9 | 9/0 (0) |
35 ± 15 (0) |
70 ± 8 (0) |
177 ± 3 (0) |
CII | 4 ± 1 (0) |
Tennis | 4 | 0/4 (0) |
24 ± 3 (0) |
58 ± 4 (0) |
168 ± 7 (0) |
CI | 3 ± 1 (0) |
CrossFit | 11 | 8/3 (0) |
31 ± 7 (0) |
70 ± 12 (0) |
176 ± 7 (0) |
CIII | 4 ± 1 (0) |
Overall | 51 | 38/13 (0) |
29 ± 11 (0) |
68 ± 10 (2) |
175 ± 6 (2) |
- | 4 ± 1 (4) |
Age (years) |
Class | N | HR (bpm) |
HRV (ms) |
QRS- duration (ms) |
ST- level (mm) |
QT- interval (ms) |
QTc- interval (ms) |
---|---|---|---|---|---|---|---|---|
≤35 | C | 39 | 83 [72; 91] |
33 [26; 47]*** |
95 [85; 104]*** |
0.00 [-0.08; 0.08] |
345 [326; 364] *** |
399 [378; 422] ** |
CI | 8 | 77 [66; 91] |
32 [31; 38]*** |
90 [88; 100]* |
0.04 [-0.04; 0.11] |
358 [343; 378] |
400 [385; 425] |
|
CII | 10 | 86 [72; 103] |
29 [21; 62]** |
93 [90; 105]** |
-0.03 [-0.07; 0] |
343 [320; 350]** |
398 [380; 423] |
|
CIII | 21 | 83 [76; 89] |
36 [26; 50]*** |
95 [85; 105]*** |
0.02 [-0.11; 0.07] |
340 [325; 361]** |
394 [377; 416]** |
|
>35 | C | 12 | 79 [71; 94] |
25 [16; 65]** |
93 [85; 100] ** |
-0.04 [-0.11; 0.07] |
340 [330; 368] |
412 [394; 414] |
CI | 0 | - | - | - | - | - | - | |
CII | 5 | 93 [73; 101] |
16 [11; 64] * |
90 [85; 100] |
-0.03 [-0.16; 0.04] |
330 [328; 378] |
414 [413; 426]§ |
|
CIII | 7 | 75 [68; 91] |
28 [17; 68] * |
95 [85; 104] * |
-0.07 [-0.13; 0.07] |
340 [333; 364] |
399 [382; 410]§ |
4. DISCUSSION
This study performed an initial investigation of features characterizing ECGs acquired through wearable sensors in supposedly healthy athletes during the pre-exercise phase and suggests a preliminary set of normal ECG feature values for pre-exercise ECGs of athletes acquired through a wearable sensor. Given the limited population sample size, these values are expected to adjust with time, thanks to results obtained with future studies. However, the value of this study relies on the fact that it demonstrated the need for reference values by highlighting differences between ECG features obtained when performing the 12-lead ECG test and those obtained from pre-exercise ECG. Suggested preliminary values were obtained by analyzing ECG recordings of 51 healthy athletes, all belonging to the Sport Database [15] and the Cycling Database of the Cardiovascular Bioengineering Lab of the Università Politecnica delle Marche (Ancona, Italy). Overall, these databases include 138 ECG tracings from 93 athletes. Excluding the multiple acquisitions for the single healthy athletes (19 ECG tracings in total), most of the recordings, however, could not be included in this study because 16 ECG tracings belong to two not healthy athletes and/or because the pre-exercise phase is not available (52 ECG tracings). The wearable sensor used to acquire the ECG was the chest strap BioHarness 3.0 by Zephyr (www.zephyranywhere.com), a device that previously proved to provide a reliable ECG [25]. ECG acquisition was performed on the training field immediately before a training session. All sports practiced by the athletes considered here belong to class C and thus were characterized by a high dynamic component [10], which associates with great cardiovascular load during exercise and high cardiac remodeling [10, 11, 26].
Preliminary values were provided for a set of ECG features (namely HR, HRV, QRS-duration, ST-level, QT interval, and QTc-interval) that are likely abnormal in case of major cardiac complications that may lead to SrSCD. Besides HR and HRV, all features refer to the electrical activity of the ventricles. No ECG features related to the atrial activity were considered since they are usually associated with non-lethal cardiac complications and their identification requires correct segmentation of the P wave, which is a low amplitude wave that is not always reliably identified in ECG recordings acquired by wearable sensors.
For ECGs analyzed here, which were single-lead tracings acquired before the training session when an athlete is typically standing and possibly moving, known reference values [12, 23, 24] normally used in clinics for resting 12-lead ECG cannot be strictly applied. Nevertheless, a comparative analysis was performed in order to obtain some insights into the athletes’ health and his/her adaptation to physical exercise (Table 2). Pre-exercise HR was not statistically different from resting HR reported in the literature; given the different acquisition conditions, this finding confirms that athletes tend to be bradycardic with respect to the general population [12, 27, 28], a phenomenon that is considered a physiological adaptation to the sport. A decreased HRV was observed in athletes; this finding is due to the fact that pre-exercise HR represents an accelerated rhythm for an athlete (i.e., the same athlete would have a lower HR in resting conditions), and HRV is known to decrease with increasing HR [29]. QRS-duration in athletes was found to increase significantly; QRS duration is an HR-independent feature, and its increment may be due to an increment of the heart size, known as athlete's heart [12], which represents the result of a complex of structural, functional and electrical cardiac remodeling in subjects practicing dynamic sports. Eventually, QT-interval tends to shorten in athletes; however, QT-interval is HR-dependent, and its shortening among athletes is statistically less significant if we consider its corrected value that is the QTc-interval.
The main limit of the present work is the small size of the study population, which makes the suggested set of normal pre-exercise ECG feature values preliminary and applicable only to athletes practicing sports in CI, CII, and CIII (Fig. 2). Surely, future ECG acquisitions on athletes are needed to validate the suggested values and provide new normative values for sports in classes AI to AIII and BI to BIII. Still, we believe that, although preliminary, the suggested set of pre-exercise ECG values can contribute to frequent monitoring of athletes’ health and, possibly, to contrast SrSCD.
According to this preliminary set, one of our athletes (subject number 5 of the Sport Database [15], male, 56 years old practicing jogging with no previous history of cardiac disease) had three ECG features (ST-level=0.12 mm; QT-duration=310 ms and QTc-duration=375 ms) out of the ranges identified in Table 2. Consequently, he was advised to undergo further clinical evaluations that, once performed, allowed diagnosis of the asymptomatic short-QT syndrome, a rare syndrome that is associated with an increased risk of sudden cardiac death [7, 30]. In general, ECG analysis from wearable sensors should be suggested for the general population of athletes (who do not necessarily perform standard 12-lead ECG test with regularity); reference pre-exercise ECG values may help to identify athletes who may develop cardiac complications and thus, need further standard clinical evaluation.
At the best of our knowledge, athlete’s ECG and exercise-related cardiac adaptation have been investigated on athletes possibly differing for ethnicity [13], practiced sport [14, 26], and sex [26], using rest or stress standard 12-lead ECGs [12] and not pre-exercise ECGs obtained through wearable technologies. Cardiac monitoring through wearable devices during athletic training mainly relies on HR and HRV analysis due to the simple, inexpensive, and time-efficient technologies (also by photopletismography) able to reliably measure HR series [27, 28]. Only a few studies proposed cardiac risk assessment algorithms through wearable devices based on the evaluation of the QT interval and HRV [7, 28] on a few athlete and non-athlete subjects.
Finally, we would like to stress that definitive reference values should be determined by many athletes after adjusting for their age, sex, amount of training, type of competition, and other factors. However, all reference values in medicine have been obtained step by step by continuously adjusting the values as more data were made available. The work proposed here represents an initial investigation of athletes’ ECGs acquired by wearable sensors during the pre-exercise phase, and proposed values are intended to be preliminary and will likely be adjusted when other databases of ECG acquired in the pre-exercise phase will be available. Currently, as far as we know, our database represents the only available database of this kind. We believe that this preliminary study may raise awareness of the need to perform these acquisitions in order to obtain more and more accurate reference values useful to large-scale prevention programs fighting SrSCD.
CONCLUSION
This study demonstrates the need for normal reference values for pre-exercise ECGs acquired through a wearable sensor from supposedly healthy athletes and suggests a preliminary set of ECG feature values in athletes practicing sports in class C. This preliminary set of values represents an initial tool to support monitoring of athletes’ health potentially before each training session in order to minimize the occurrence of SrSCD.
LIST OF ABBREVIATIONS
SrSCD | = Sport-related Sudden Cardiac Death |
ECG | = Electrocardiogram |
Max O2 | = Maximal Oxygen Uptake |
MVC | = Maximal Voluntary Contraction |
mRR | = Mean RR Interval |
HR | = Heart Rate |
HRV | = Heart-rate Variability |
mBeat | = Median Cardiac Beat |
Rp | = Peak of the R Wave |
QRSon | = Onset of the QRS Complex |
QRSoff | = Offset of the QRS Complex |
Tp | = Peak of the T Wave |
Toff | = Offset of the T Wave |
ETHICS APPROVAL AND CONSENT TO PARTICIPATE
Data collection for this study was approved by the institutional (Università Politecnica delle Marche, 60131 Ancona, Italy ) expert committee.
HUMAN AND ANIMAL RIGHTS
No animals were used in this research. All human research procedures were followed in accordance with the ethical standards of the committee responsible for human experimentation (institutional and national) and with the Helsinki Declaration of 1975, as revised in 2013.
CONSENT FOR PUBLICATION
All athletes gave their informed consent prior to data acquisitions.
AVAILABILITY OF DATA AND MATERIALS
The data related to Sport DB [15] and Cycling DB used in this study are available from the corresponding author [M.M] upon request.
FUNDING
None.
CONFLICTS OF INTEREST
The authors declare no conflict of interest.
ACKNOWLEDGEMENTS
Declared none.