All published articles of this journal are available on ScienceDirect.
Review On Machine Learning and Deep Learning-based Heart Disease Classification and Prediction
Abstract
Coronary Heart disease is the major factor for people’s deaths throughout the world, and it is necessary to detect and predict the disease in the earlier stages because time plays a vital role to save the coronary patient. From this paper, the authors can conclude that authors have used most of the machine learning and deep learning ensemble algorithms so that they can predict heart disease at the early stage so that the patient’s life can be saved.
1. INTRODUCTION
Heart Functioning is essential for the maintenance of normal systematic function of human lifestyle. Heart dysfunction can lead to a lot of problems in day-to-day life leads to fatigue, and dysfunction of the heart leads to heart failure and the mortality rate is going to be increased in case the left ventricular ejection or if there are any slight changes in that left ventricular part it leads to life-threatening process for human beings and leads to lifetime therapy and should lead to lifelong medication so it is necessary to predict the cardiovascular diseases the earliest form. In this paper, we analyze the data and feature extraction abilities by using deep learning and proposed convolution neural networks and video clips for cardiomyopathy classification. In this, we need to create high-standard videos that represent grayscale images without functioning or preprocessing and creating optical framework. Then need to evaluate these metrics of performance with the help of three types of trained models, Normal, Low ejection & Arrhythmia.
Echocardiography is the most highly used throughout the world and available readily for imaging techniques to access the heart functioning and structure, a combination of image acquisition, with high temporal and without making use of ionizing radiation, echocardiography is the main backbone for heart-related images. Even echocardiography is well suited for diagnosing cardiovascular and even very suitable for various applications of deep learning and machine learning techniques.
This paper analyzes the uncommon component extraction capacities of profound learning and proposes a convolutional network combined with Video Clips for cardiomyopathy order. The goal is to achieve a superior profound learning model for evaluating cardiovascular capacity in echocardiogram recordings and recognize the information video that has a place in the accompanying three classes: Normal, Low Ejection Fraction, and Arrhythmia. We execute a framework that screens subsampled cut exercises to accomplish more exactness utilizing the Convolution Neural Network calculation and plan a web application where clients can enter echocardiogram recordings and get their characterization results.
2. LITERATURE SURVEY
2.1. CEP4HFP – Complex Event Processing for Heart Failure Prediction
In this paper, the author proposed for cardiovascular disease patients to extract the health parameters and they are controlling them from a far distance. Secondly, they analyze these data and update the health record system during runtime automatically by making use of IoT devices like wearable sensors, which is going to extract all health parameters from cardiovascular disease patients and monitors them remotely without changing the actual values and. In this paper, they introduced a novel methodology called CEP4HFP for predicting heart disease.
2.2. Identification of Cardiovascular Disease from ECG Images Using Machine Learning and Deep Learning
In this paper, the author used the transfer learning approach, which was investigated by making use of the low extension prearranged Alex net. Secondly, they defined a convolutional neural network diagram that can detect the dysfunction of the heart; thirdly, affirmation CNN is utilized to extract the features of machine learning algorithms, mainly KNN, SVM, DT, NB & RF. According to this experiment, the performance metrics for the proposed models and the performance analysis accomplished accuracy of around 98.22%, precision is 98.31%, F-score 98.21% and recall is 98.22%.
2.3. Standard-Based Widespread Heart Controlling Method using Narrowband IOT Queuing Model Analysis in IEEE 1451 Standard
In this paper, the author has used IEEE 1451 sensors, smart sensor, Heart monitoring, NB-IoT, using the band wrist, which is tied to a patient. It will be monitored remotely through mobile, the ECG, and it is connected through wireless and wired sensors [3].
2.4. Classification of Heart Audio by making use of Deep Learning
In this paper, the author has used a new technology called phonocardiogram (PCG) apart from ECG, this technology is used to detect abnormalities in the heart with the help of phonograms when the heart audio is collected which contains interleaved noises with the help of PCG the main motto of this PCG(Phonocardiogram)is to find the systolic & diastolic pressures in the heart in terms of patterns of the sound and detecting the heart disease [4].
2.5. Atrial Fibrillation Detection using Electrocardiogram by using Deep Learning
In this paper, the author has described atrial fibrillation (AF) as an abnormality related to cardiovascular. The author has described classifying the ECG signals by making use of LSTM, i.e., Long short-term Memory, and Gradient Recurrent Unit(GRU), and classified into three forms i.e., Normal, Atrial fibrillation and Rhythms. Physio net Challenge 2017 is the dataset used to study this and the obtained results of LSTM & BiLSTM are compared by making use of SVM (support vector machine), the result shows that the use of LSTM has improved performance when compared to BiLSTM & SVM. For classification of accuracy, normal, AF & Rhythm show the result as Norma is 96.92%, AF is 97.36%, and Rhythm is 96.39%, respectively. By making use of LSTM, the overall accuracy has been improved to 96.94%, which has developed a technology having immense application in the medical field [5].
2.6. Investigating the Preprocessing Methods in ECG Analysis
Nowadays the number of fitness freaks has been increasing, for this, they need authentication for protecting their private data like biometrics, face recognition, and Voice Recognition, because they are using it as login credentials but have privacy issues. In this paper, the author introduced the smart bands that can track cardio movements and all related to electrodermal authentication and gathered all physiological signal information from the smart band, and which is used to create multimode & multisensory authentication systems. This smart band enables to use of new applications without the intervention of hardware devices. In this research, the authors explored multimodal physiological authentication data like (Biometrics, Face Recognition) using the feature-based traditional peer-to-peer deep learning system. By collecting the multimodal physiological data from the featured related peer-to-peer machine learning systems to classify the CNN & LSTM system and achieved a 9.3% error rate and even performed the authentication function [6].
2.7. Heart Controlling Real-time System using Energy-Efficient Cloud Internet of Medical Things
New advancements in gadgets that are wearable and the Internet of Medical things (IOMT) permit constant observing and recording of electrocardiogram (ECG) signals. Be that as it may, ceaseless observing of the signals from ECG and trying to use low-power gadgets that are wearable. Because of energy and memory restriction [7], hence, in this research, a novel methodology has been proposed using energy-productive techniques for persistently maintaining the heart rate by making use of wearable gadgets. The proposed methodology has three unique features made out of three layers.
(a) Noise -Artifact identification layer to rate the nature of ECG signals.
(b) Normal-Abnormal beats arrangements, this layer is used to identify the abnormalities in ECG signals.
(c) An abnormal heartbeat characterization layer, to recognize illnesses ECG signals [8].
Besides, the circulating multi yield convolutional neural network(CNN) technology is utilized to diminish the strength utilization and inactivity between the edge/haze cloud. This technique gives an accuracy of 99.2% on the notable MIT-BIH Arrhythmia dataset. Moreover, the proposed physiology accomplishes 7* more energy proficiency contrasted with cutting edge work [9].
2.8. Heart Rate Estimation using Graph-based Denoising for Respiration during Sleep in the Thermal Video
Quality of rest is a fundamental human necessity for success, yet the absence of sleep will lead to overall health issues. An abnormal sleep pattern will lead to sleep apnea, because of this, people few times quit breathing during set down resulting in surprising basic signs, being explicit breath rate and pulse [10]. While a giant struggle has been made for imperative symptom checking structure during resting time, existing methodologies need to convey the capacity for unwieldy and intrusive structures and relentless quality for client level, non-meddlesome frameworks [11]. To defeat any boundary, practicality and accuracy work with smart clinical benefits using the Internet of things (IoT). In this research, the author proposed a critical sign evaluation structure during sleep, and pictures are captured through a camera. The structure takes a warm picture grouping of a resting subject and subsequently processes the facial features inside the picture. For principal signal extraction [12]. Basically, using the intrinsic graph architecture. Among the subregions of the facial features, the author proposed an outlined-based spatial passing sign and denoising plan and proposed an exploratory system to reduce denoising and introduced the cameras that subjects structure beats which are bleeding-edge non-noisy crucial [13].
2.9. Classification of Electrocardiogram based on Deep Learning Approaches
As of late PC supported conclusion techniques have been generally taken on to help specialists in illness find settling on their choices more solid and blunder-free. Electrocardiogram (ECG) is the most generally utilized, painless analytic apparatus for researching different cardiovascular illnesses. In actuality, patients experience the ill effects of more than each coronary illness in turn [14]. Along these lines, any practical mechanized coronary illness analysis framework ought to recognize the various heart infections found in the signals emitting from ECG. In this research, the author proposes a clever and profound learning strategy for the multilabel arrangement of signals from ECG [15]. The proposed methodology precisely recognizes two marks of an ECG Signal relating to eight morphological anomalies of the heart and it is even for a normal heart condition. Additionally, discovered the idea of profound gaining models and applied them to analyzing computerized coronary illness. Therefore, in this article, we recognize a logical computerized reasoning (XAI) system for ECG characterization utilizing class enactment maps taken from high fraud CAM. This proposed methodology needs to train Convolutional Neural Networks(CNN) by embedding ECG grids. By examining this, we need to prepare CNN by taking single ECG signal information and this point is sufficient for organizations to get the familiar ECG point with multi-labeled data (that can identify numerous cardio illnesses simultaneously) [16]. During grouping, we need to apply the result probability of the SoftMax layer of CNN, which acquired the ECG signals with multilabel. In this research, the novel method is used with 6311 ECG records and tried with 280 ECG records at the time of testing this process, the model accomplished and accuracy of 96.2% and hamming loss is 0.037% precision is 0.986%, recall is 0.949 & F1-Score is 0.967% [17].
2.10. Findings of Heart Disease by Making use of ECG Images
Cardiovascular sickness (heart infection) is the main source of death around the world, as it can be anticipated prior and saved many lives with the help of ECG (Electrocardiogram). It is a cheap and harmless device for estimating the activity and electrical signals in the heart and it is utilized to recognize heart attacks [18]. In this research, the force of profound learning strategies is utilized to anticipate four significant cardiovascular anomalies i.e., strong heartbeat, myocardial localized necrosis, history of myocardial dead tissue and ordinary individual classes using the ECG picture and the data of cardiovascular patients [19]. To start with, in exchange, the learning approach was researched utilizing the less scaled prepared profound brain contents to squeeze net, Alex net secondly, another convolutional neural network(CNN) design is proposed for cardiovascular irregularity expectation [20] third in advance of the referenced preprepared models, and the proposed CNN architecture is utilized as a component extracting device for customary AI calculation’s, to be specific, other machine learning algorithms as per the outcomes the measurement is acquired in the accuracy of 98.32%, precision is 98.28%, recall is 98.44% F1 score is 98.26%. After using CNN architecture, it acquired accuracy around 99.78% utilizing the NB calculations [21].
2.11. Detecting Coronary Artery Disease by using the Phonocardiogram & Ensemble Transfer Learning
Traditional AI has made ready for a basic, reasonable, painless way to deal with coronary conduit illness (CAD) discovery utilizing phonocardiogram (PCG) [22]. It passes on an extension to investigate the improvement of execution measurements by a combination of gained portrayals from profound learning. In this research, the author proposed a novel method by using MKL combination utilizing profound embedded with CNN. The novel method MKL tracks down. The ideal part mixes by expanding the likeness with the best portion and limiting the overt repetitiveness with other premise bits [23]. Tests are performed on around 960 phonocardiogram ages from 40 CAD and 40 different typical subjects. The embedded achieved a greater precision of around 89.52% with a kappa of 0.7852. Afterward, that combination of hand-tailored highlights utilizing the novel MKL method gives an accuracy of around 92.21% with kappa 0.8328. The review shows the capacity for the advancement of an exceptionally precise CAD discovery framework by utilizing a simple-to-get, harmless PCG signal [24].
2.12. Blood Pressure measured by using Advanced Noninvasive Techniques
Hypertension, or raised circulatory strain (BP), is a marker for the vast majority of cardiovascular sicknesses and can prompt perilous circumstances like cardiovascular breakdown, coronary corridor illness, and stroke. A few strategies have as of late, been proposed and examined for harmless BP observing. The rising craving for telemonitoring arrangements that permit patients to deal with their circumstances at home has to spend advancements in BP checking strategies [25]. In this research, the author presents the new advancements in
painless pulse checking arrangements accentuating clinical approval and compromise between accessible methods. We present the ongoing BP estimation procedures with their hidden working standards [26]. New encouraging evidence of idea studies are introduced and ongoing demonstrations and AI approaches for further developed BP assessment are summed up. This helps the conversation on how new BP screens ought to be assessed to deliver new home checking arrangements in the wearable structure factors. Lastly, we examine unsettled difficulties in making advantageous, solid, and approved BP observing arrangements [27].
2.13. Real-time Heart Disease Prediction System using Multilayer Perceptron
Heart attack, epilepsy, and local necrosis are the most widely recognized heart disease infections and have the highest death rate on this planet. Cardio absconds are unrecognized at the beginning phase because of the illogical expenses of the test accessible [28]. Consequently, a quick, continuous, and solid framework it predicts the possibility of having a coronary illness is a streamlined way of examining the neural architecture utilizing the multilayer perceptron(MLP) for forecasting framework. The trial examination brought about accuracy is 85.75% [29]. It contrasted with past examinations when the expansion was around 12-13%. A basic web application apparatus is likewise evolved by utilizing the testing process of the Python forecasting framework [30]. The exploration pursues making a conceivable apparatus for clinical experts as well as ordinary citizens.
2.14. Using Hybrid and Data Mining Classification Techniques to Predict Cardiovascular Disease
Coronary illness forecast is recognized as a more convoluted task in clinical sciences and consequently needs emotional and timely precautions that can save coronary patients [31]. In this research, the author proposed taking the history of family (i.e., hereditary calculations and taking back propagation procedure to predict the coronary illness forecast. Today, the medical field has made some amazing achievements to sort out illnesses [32]. Making use of the hereditary dataset combined with the backpropagation algorithm, the author
proposed a novel method to predict heart disease, and to achieve better accuracy (Examples and relations) connected with coronary illness from a past coronary illness dataset record. It can settle confounded questions for identifying coronary illness and hence help clinical experts to pursue brilliant clinical choices that conventional choice emotionally supportive networks could not [33, 34]. Giving productive medicines can assist with diminishing the expenses of treatment.
2.15. Coronary Vascular Disease Screening System
This paper depicts a disconnected PC-based framework to order typical and strange heart sound signs from the heart strong sound document [35]. The framework peruses the chosen heart sound sign naturally, portions the heart sound signs into tests, removes the component of each example utilizing the cross-relationship strategy, and groups the examples utilizing the progressive multi-facet perceptron network. MATLAB interface is utilized to make the framework bridge in the PC stage [36]. The architecture yields a high level of screening precision (96.3%), recall (92.59%), and accuracy (94.44%) [37].
2.16. Predicting Coronary Vascular Disease at Initial Stages using Machine Learning
Anticipating and identifying coronary illness has forever been a basic testing task for medical services experts. Clinics and different centers are offering costly treatments and tasks to treat heart sicknesses [37]. Along these lines, anticipating coronary illness at the beginning phase will be helpful to individuals all over the planet so they will make important moves before getting extremely. Coronary illness is a critical issue lately; the fundamental justification behind this infection is the admission of liquor, tobacco, and the absence of actual activity [38]. Throughout the long term, AI shows compelling outcomes in deciding and forecast from the expansive arrangement of information delivered by the medical care industry [39]. A portion of the directed AI strategies utilized in this expectation of coronary illness are counterfeit brain organization (ANN), choice tree (DT), arbitrary woods machine learning algorithm RF(Random Forest), SVM support vector machine, NB(Naïve Bayes) and the exhibitions of these calculations are summed up.
2.17. Cardiovascular Disease Prediction using big Data Approach
Throughout the course of recent decades, heart attacks have been the reason for human demise. Thus, the early detection of coronary illness & consistent observations can lessen the chances of death. In this research, the author has proposed making use of AI & Apache-spark technology to predict cardiovascular disease to achieve the better accuracy.
2.18. Classification of Cardiovascular Disease Prediction using Data Mining
Nowadays, massive deliberation of information yields and is gathered in each case of time. Along these lines, investigating them is the hardest assignment to do. This colossal volume of information has been created from dissimilar sources like medical services, virtual entertainment, business applications, fabricating ventures, and more [40-43]. Medical care assumes an urgent part in data mining and protecting illnesses at a crude stage is particularly essential. Coronary illness explicitly suggests a state of the heart that agreements or discourages veins, which brings about torment in the chest and cardiovascular failure [44]. This paper stresses the finding of heart illnesses at a crude stage with the goal that it will prompt a fruitful fix of the infection. In this paper, successive thing digging is utilized for separating the properties, and different information mining arrangement techniques like machine learning algorithms “Decision tree” order, “Naïve-Bayes” grouping, Support Vector Machine characterization, and “K-Nearest Neighbor” are utilized with the assurance that the shielding sickness in a beginning phase when it is very well may be dealt which is protected [44-55] (Fig. 1).
Evaluating the system:
In this research, the datasets used are benchmark. Echocardiogram videos are converted into frames by Data Preprocessing techniques that is feature extraction is done once the video datasets are converted into frames which are randomly selected for the input process, and then there is 80% of the data is used for training and 20% of the data is used for testing the data by making use of the CNN Module which consist of an Input layer, Hidden layer, and output layer in which the RELU activation function is used once the testing data is done it is used for classification the used input frames and is going to display the classification as a) Normal- 60-70 HB/min b) Low-ejection-40-50HB/min c) Arrhythmia -Below 30 HB/min (Fig. 2).
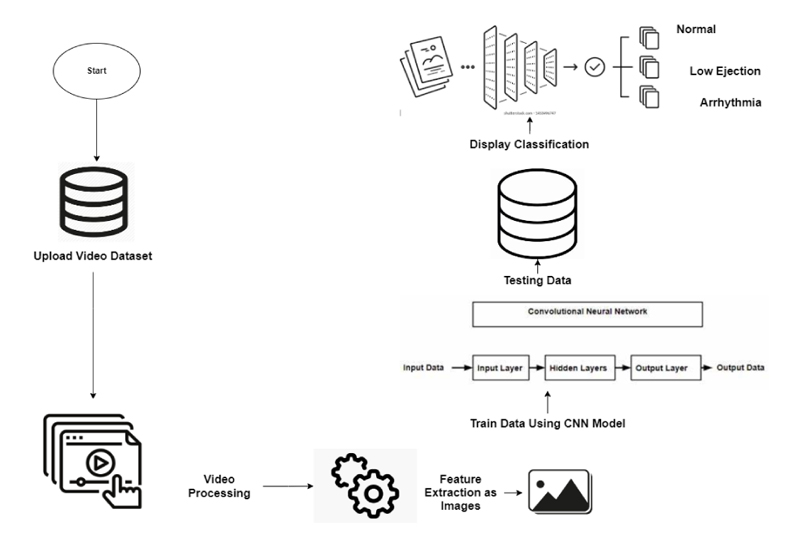
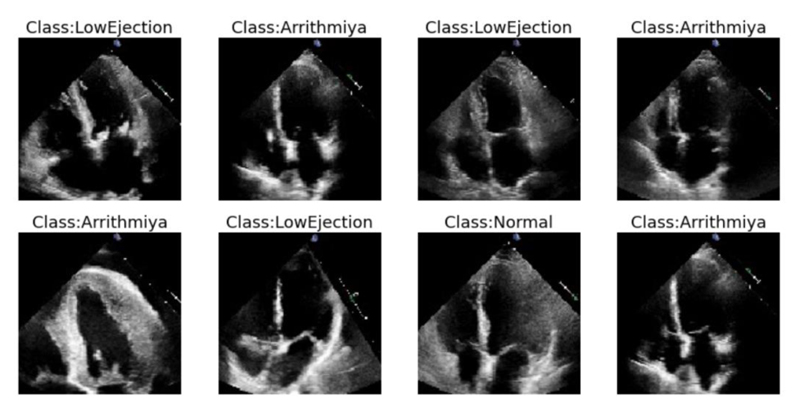
The above figure shows the samples of echocardiogram video data sets which are converted into frames as low normal and Arrhythmia.
2.19. Experiment
2.19.1. Data Pre-processing
In the data preprocessing, the input is taken as video datasets as shown in the below figure, with the video name and tags.
In the mentioned method, different videos are used in which the videos are preprocessed videos are converted into frames. This dataset contains 1024 patients with three different classes.
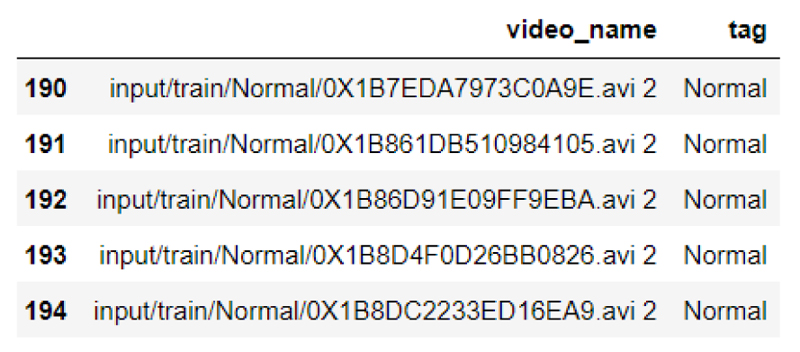
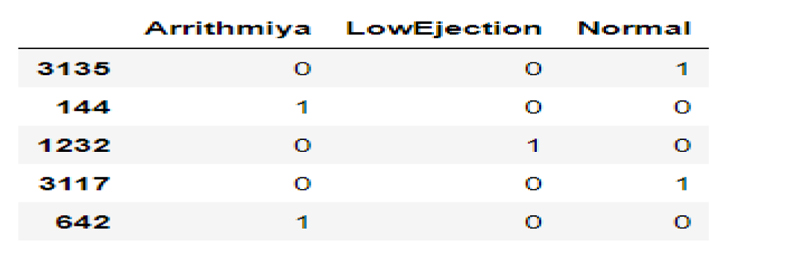
a) Normal b) Low-Ejection c) Arrhythmia. Fig. (3) depicts the different images that have different samples that have Normal, Low-ejection, and Arrhythmia.
At the data preprocessing stage, once the video datasets are converted into frames, they have been trained and tested in which 80% of the data is used for training purposes, and the remaining 20% of the data is used for testing purposes as shown in Figs. (4 and 5).
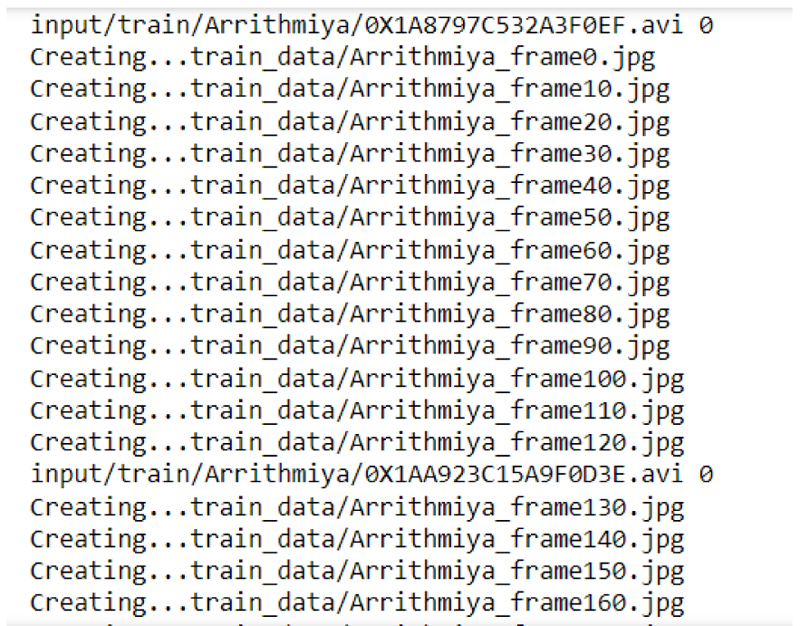
2.19.2. Data Splitting
After the data preprocessing stage, the data splitting is done in which around 80% of the data is used for training purposes and the remaining 20% is used for testing purposes as depicted in Fig. (6).
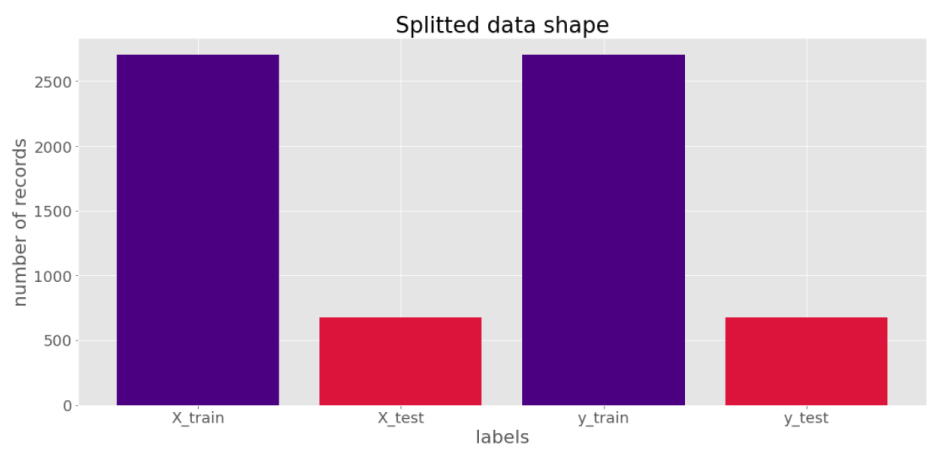
Once the frames have been trained data splitting process can be done in which 80% of the data is used for training and 20% of the data is used for testing purposes, Data splitter graph has been shown in the graph.
Using the Convolutional Neural Network the data has been layered into different layers as shown in the Figure in which the classification of different convolution layers can be seen.
After undergoing all the processes, the data were trained and tested by making use of the CNN module as shown in the figure.
3. SUMMARY
From the above sections, different authors have proposed different methodologies for heart disease prediction considering the above, most of the authors predicted and used distinct algorithms using deep and machine learning methods to predict cardiovascular disease.
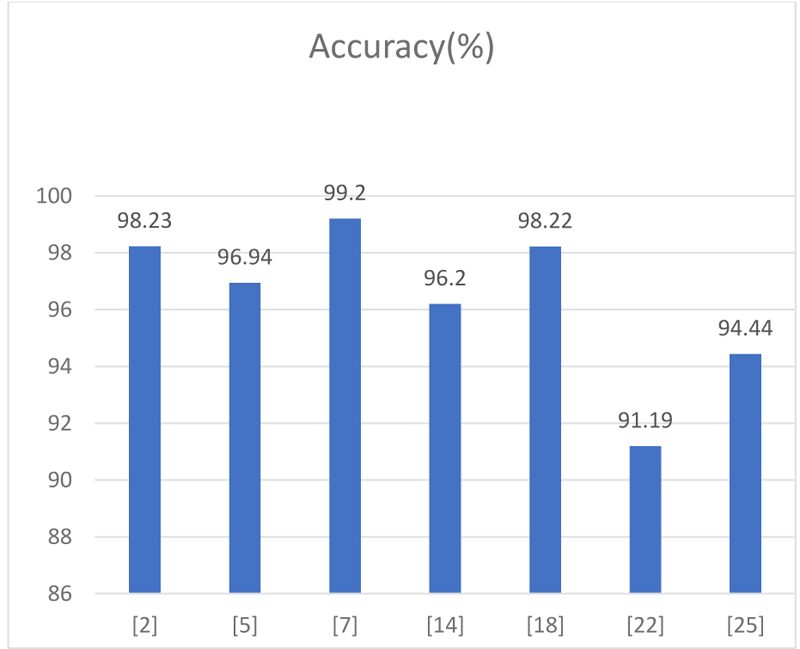
The authors even introduced different devices like wrist band, hand-carried devices like wearable to predict the heart disease.
Some other authors have used ECG and video datasets for extraction of the features of the images and predict heart disease. Some of them even predicted by making use of different ensemble algorithms of machine learning like NB, RF, SVM, regression, and classification methods to predict cardiovascular disease.
Few authors used Big-data techniques like Hadoop and artificial techniques to predict the heart disease.
Some authors discussed by making use of Different Numerical datasets like age of a person, cholesterol level, and blood pressure, and even making use of family history helps in predicting heart disease.
It is better to make use of modern technologies to predict heart disease and can save the patient’s life at the earliest stages.
From the above graph (Table 1, Fig. 7), we have taken the accuracy rate of different papers proposed by the authors analyzed and depicted in the graph.
Accuracy(%) | |
---|---|
2.3 | 96.2 |
2.4 | 98.23 |
2.5 | 91.19 |
2.7 | 85.71 |
2.9 | 94.44 |
CONCLUSION
Coronary Heart disease is the major factor for the people’s deaths throughout the world, and it is necessary to detect and predict the disease in the earlier stages because time plays a vital role to save the coronary patient, which we call a “Golden hour” is very much necessary we need to get some more advanced technologies to predict the heart disease so that we can save the patients as early as possible. Therefore we can reduce the death rate treatment at the right time. From this paper, we can conclude that we have used most of the machine learning and deep learning ensemble algorithms so that it can predict heart disease at an early stage so that the patient’s life can be saved.
LIST OF ABBREVIATIONS
PCG | = Phonocardiogram |
AF | = Atrial fibrillation |
GRU | = Gradient Recurrent Unit |
ECG | = Electrocardiogram |
IOMT | = Internet of Medical things |
CNN | = Convolutional Neural Networks |
RF | = Random Forest |
CONSENT FOR PUBLICATION
Not applicable.
FUNDING
None.
CONFLICT OF INTEREST
The authors declare no conflicts of interest, financial or otherwise.
ACKNOWLEDGEMENTS
Declared none.