All published articles of this journal are available on ScienceDirect.
A Novel Approach to Information Security in Medical Sensor Networks
Abstract
Objective:
Preservation of patient’s medical information in health care industries under Medical Sensor Networks (MSN).
Methods:
This paper proposes a novel key management technique known as k- secure with FBKM, which generates a robust key to allow communication between sensors present in the Body Sensor Units (BSU) and Body Central Unit (BCU). This proposed work strengthens the FBKM technique which is placed between BCU and the point accessible to medical experts at a remote place in the overall health care monitoring environment.
Results:
The FBKM technique has proved its success in authentication and security by improving genuine acceptance rate, false rejection rate, and declining false acceptance rate.
Conclusion:
The k- secure with FBKM scheme enhances the performance of the existing FBKM scheme in Medical Sensor Networks.
1. INTRODUCTION
The capability to observe a patient’s health status physically is imperative in critical situations such as heart attacks, strokes, natural calamities, and during wars. The contemporary developments in health care industries have resulted in sensors that can provide proper observation of patients [1]. Medical sensors on a patient’s body are interconnected to gather information related to health. In a crisis, medical experts can set up sensors on the injured patient which can help examine or observe essential crucial symptoms.
As MSNs deal with personal medical information, protecting them as well as communicating that information over the wireless medium is equally challengeable. External or internal attackers can modify the original medical data, and the lack of insufficient security features may violate the patient privacy, which can result in an erroneous diagnosis and treatment [2]. Health Insurance Portability and Accountability Act (HIPAA) is insisting on the importance of protecting delicate information related to the legal aspects of physical conditions [3]. Further, urgent situations generally have some amount of pressure coupled with people, for example, the golden hour as in the occurrence of heart attacks [4].
Sensors depend on cryptographic keys to protect medical data communication. Security in MSN adapts to protect medical information from illegal access, revelation, disruption, modification, and destruction. Key generation and dissemination in sensor networks typically involve a certain form of pre-implementation. SPINS [5] and LEAP [6] are some of the protocol suits to perform key distribution in sensor networks. On the other hand, the gradually growing size of Medical Sensor Networks, the requirement of sensor node placement in managing crises, and conventional methods may include substantial latency during communication of medical data between source and destination.
The following observations have motivated the development of the proposed work on medical sensor networks.
- Significant additional infrastructure would be required to make MSN transmission secure and precise.
- There is a need to ensure that the patient's secure information is obtained only through each patient's committed MSN system and is not mixed up with other patient's information. Furthermore, the information created from MSN ought to have secure and restricted access.
- As MSNs are resource-limited in terms of power, memory, communication rate, computational capacity, and security, the arrangements proposed for other networks may not be applicable.
- Security requirements like privacy, confidentiality, authentication, integrity, and freshness of information together with accessibility and security need to be accorded high priority in MSN.
- Several security-related hardware-driven issues such as interoperability, intrusion into privacy, validation of sensors, information consistency, obstruction, and information management needs to be taken into account in MSN system development.
- Human-driven issues such as cost, need for continuous monitoring, constrained exploitation, and reliable performance are additionally considered while developing robust MSN.
This paper describes a novel key management scheme named as k- secure with FBKM, which is implemented between Body Sensor Units and Body Control Unit a.k.a the local loop. The proposed scheme has been developed to strengthen the security level in the local loop itself. Further, the proposed scheme integrates Fuzzy based Bio-Key Management (FBKM) technique [7] to provide a promising and robust security level in data communication in Medical Sensor Networks.
The paper is organized as follows: Section 2 describes the related work, Section 3 depicts the system model, Section 4 displays the experimental results. Section 5 concludes the paper.
2. MATERIALS AND METHODS
In this section, various research works related to information security in body area networks have been reviewed. ECG-IJS scheme executed between the body control unit and destination such as hospital server, caretaker or medical expert node proved its performance by providing security with fewer computational requirements as well as zero key distribution overhead [8]. K.Kalaivani & R.Sivakumar presented a Fuzzy based Bio-Key Management (FBKM) scheme for medical data communication between medical sensor network gateway and destination server or emergency service or medical expert node [7]. This algorithm strongly supports telemedicine-based applications to ensure privacy and patient safety. FBKM exploits physiological signals such as ECG signal for creating cryptographic keys. This algorithm could be realized in a plug and play manner, which means no previous key distribution is required. This scheme has made security systems stable by providing low FRR value. This scheme provides lower computation complexity and communication overhead. This efficient scheme offers security in terms of authentication, data confidentiality and data integrity. This efficient algorithm has proved its effectiveness by providing low FRR, High GAR and Low FAR, compared with ECG-IJS and PSKA algorithms.
Abdulaziz Alsadhan & Naveed Khan have proposed LBP (Local Binary Pattern) based hybrid type of cryptographic technique for WBAN, which uses EKG signals for key generation [9]. Ayan Banerjee et al. have proposed a different protocol called Physiology-based End-to-End Security PEES, which provides a protected communication network between sensors and organized medical information. PEES protocol was designed to use features extracted from ECG or PPG signal for concealed key generation, and artificially created ECG or PPG signals from procreant models to flaunt keys [10]. Carmen C. Y. Poon & Yuan-Ting Zhang proposed an approach for telemedicine-based applications. This article explores a biometric approach that uses the inherent features of a person for cypher key generation in medical sensor networks [11]. Dagtas et al. proposed a secure algorithm for key formation, to encrypt patient data from physiological sensors to medical experts or the device of movable patients [12]. Daojing He et al. introduced a technique to preserve the collected data from patients. This technique utilizes a hash algorithm based technique to attain protected transmission and in-depth data entry control [13]. Daojing He et al. discovered the safety liabilities in medical information discovery and proposed a safe and reliable protocol to ensure the purity of medical information [14]. Guanglou Zheng et al. presented an algorithm that utilizes multiple ECG feature values to breed random binary sequences with minimal latency [15]. Najeh Jammali & Lamia Chaari Fourati have proposed the physiological based scheme to accomplish secure communication between sensors [16]. Calendar Choudhary & M. Sabarimalai Manikandan presented a strong PPG based scheme for body area networks and Tele-health applications [17]. Penglin et al. proposed an approach for sensors in BAN to launch group secrets using Physical Unclonable Functions, which reduces the communication overhead [18]. Mana Al Reshan et al. developed a cryptography technique built on both the biometric and physiological signals. This new approach reveals improvement in accuracy and authentication [19]. K. Kalaivani et al. proposed an efficient bio key management technique for telemedicine-based applications [20]. Samaher Al-Janabi et al. examined the architecture of wireless BAN communication, requirements for security aspects, security threats and the major challenges based on the existing medical standards [21]. Nidhi Sharma and Ravindra Bhatt have suggested a scheme for wireless sensor network-based healthcare application exploiting the doctrines of multipath routing [22]. Xun Yi et al. proposed a concrete approach to counteract the inside attack with the help of numerous data servers to accumulate patient information [23]. Prosanta Gope and Tzonelih Hwang focussed on techniques for key security constraints in a body sensor network centred prevailing healthcare systems [24]. Karthikeyan, M.V. and Manickam, J.M.L developed an ECG signal based scheme, which is a competent device model intended to prepare confidential keys from the ECG signal parameters merged with the current secure force (SF) technique; the technique is investigated for robustness [25]. Z. Zhang et al. presented an innovative key agreement technique that agrees on adjacent nodes in medical sensor networks to distribute a public key created by EKG signals. This key agreement technique can protect information communications without any key dissemination overheads [26]. Sofia Zebboudj et al. presented a new technique for biometric parameter extraction and demonstrated the strength of the technique against vulnerabilities [27]. Pirbhulal S et al. designed a new kind of electrocardiogram based biometric feature extraction technique that utilizes Data Authentication Function for the protection of medical sensor networks [28]. D. K. Altop et al. defined a unique physiological feature extraction technique and used inter-pulse interval derived from PPG and ECG signal for key generation in body area networks [29]. H. Zhao et al. evaluated the performance of various protocols efficiently used in body sensor networks and proposed various solutions to handle demerits [30].
Based on the observations from the survey, k-secure with FBKM scheme is proposed to be implemented between sensors present in the Body Sensor Units (BSU) and Body Central Unit (BCU) in this paper. This paper proposes an innovative algorithm “k-secure with FBKM” to support medical data communication between sensors and gateway in Medical Sensor Networks. Although FBKM algorithm [7] is excellent in providing stable and secure communication, it does not ensure the originality of the data and authentication of the user in the MSN gateway node. FBKM algorithm provides a greater level of security between MSN gateway and destination point only. But in reality, there is no guarantee that the attackers would play only between gateway and destination point.
There are chances for the intruders or attackers to hack the data inside the sensor network also. Attackers may be from an internal source or external source. Attackers may snoop into data transfer inside networks, insert messages, repeat older messages and skit node uniqueness. Based on this issue, k-secure with FBKM algorithm is proposed to support FBKM algorithm. Now by incorporating these two algorithms, the telemedicine system can be made stable and secure.
3. SYSTEM MODEL
Medical Sensor Network (MSN) is an interconnection of physiological sensors that are embedded in the human body. Sensors can be ECG, EMG, glucose, blood pressure, inertial sensor etc. and are employed to collect the data periodically and send data over a conventional system to a destination point. Sensors in MSN transmit and receive data through the wireless medium.
The k- secure with FBKM algorithm which is proposed to be implemented between Body Sensor Units (BSU) and Body Control Unit (BCU) may be called a Local unit as shown in Fig. (1). Although various robust algorithms are available to ensure authentication and security between BCU and medical expert node destination or emergency mobile unit or health information servers, there is a probability for a security threat to happen in the local unit itself. Therefore, it is mandatory to protect patient data within the local unit itself.
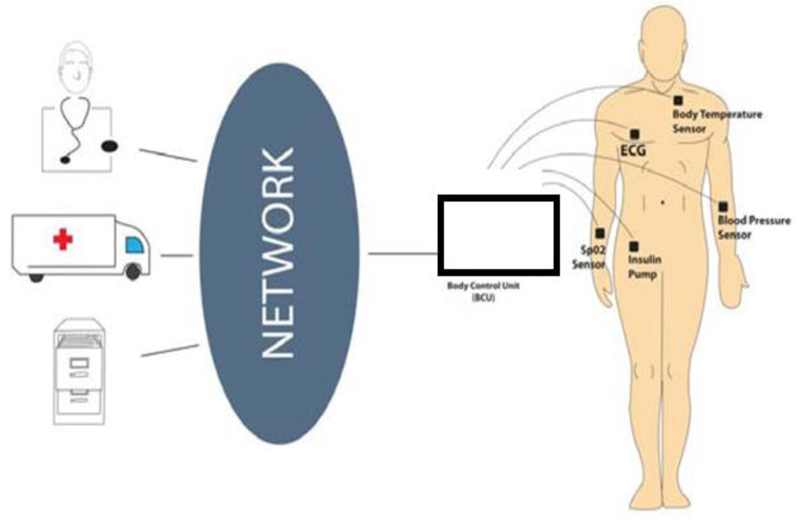
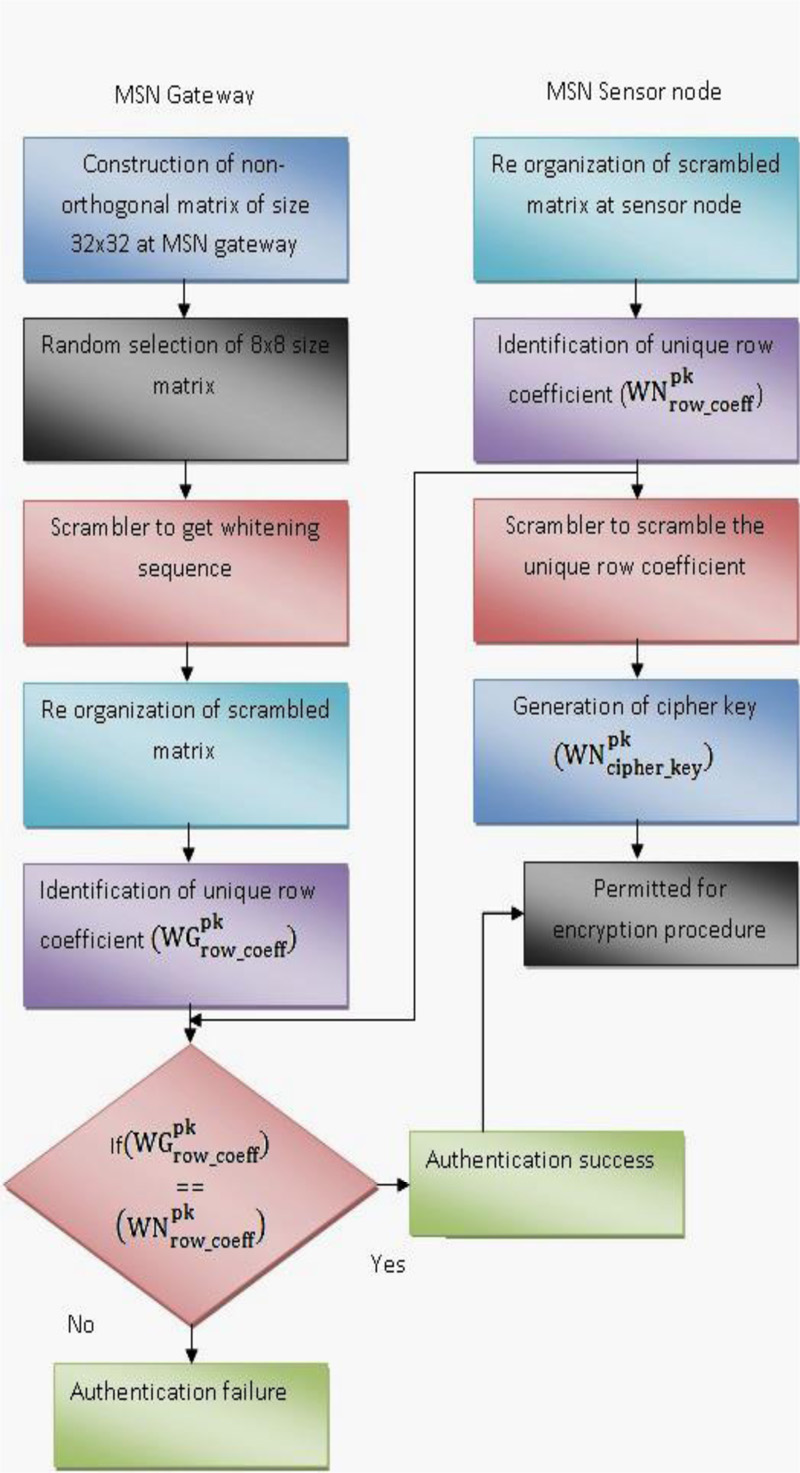
Challenges faced by the MSN are principally from rivals who can overhear something within an MSN, infuse false information, rerun older reports, and imitate original nodes. Based on these threats, k- secure with FBKM algorithm restricts the entry of unauthorized attackers into the local unit and improves authentication level from source (Patient) to destination (Medical expert node, Hospital, Caretaker, etc.).
3.1. Authentication Process
Authentication process between MSN gateway and sensor node is shown in Fig. (2) and detailed explanation is also provided.
Step 1: Body Control Unit or gateway in MSN generates a non-orthogonal matrix of 32x32 size using non-orthogonality function represented as in matrix (1). Here, non-orthogonal matrix is constructed with the elements as given in parenthesis (-1, 0, 1).
![]() |
(1) |
where n = 32.
Step 2: A small dimension 8x8 matrix is randomly chosen and is indicated in matrix (2)
![]() |
(2) |
Rows in the matrix are randomized by passing them through a scrambler. A scrambler reinstates sequences (whitening sequences) into other sequences without eliminating unwanted sequences, and as a result, it alters the likelihood of the occurrence of error-prone sequences. The scrambled matrix is sent to all MSN sensor nodes and may be represented as in matrix (3)
![]() |
(3) |
Step 3: MSN sensor node receives scrambled sent by Body Control Unit or gateway. k-secure with FBKM triggering at MSN sensor nodes.
Step 4: Initially, k secure FBKM re-organizes the scrambled matrix. Next, it identifies the row that satisfies non-orthogonal element (Total of all elements row-wise is larger than or not equal to zero), the unique pattern. The row that first satisfies the non-orthogonal factor is identified and the row id of the row that satisfies the condition is set as the unique row-coefficient (). Assume row 2 is unique, and then row- coefficient can be denoted as given below:
![]() |
(4) |
If a single row fails to satisfy the non-orthogonality factor, then the 8x8 matrix is divided into multiple 4x4 matrices and the criteria are verified. The process continues until a unique 1x8 matrix pattern is identified. There are possibilities that a single row or combination of multiple rows can form a unique pattern. Row ids of the selected rows are organized to form the set of row coefficients.
Step 5: Row coefficients are stored by the MSN sensor node. It randomizes the set by passing it through the scrambler and sends unique coefficients to the MSN gateway and is represented as in matrix (5):
![]() |
(5) |
Using the 1x8 unique pattern, alternate row data are combined to create a 4-bit cypher key used for data encryption as shown in matrix (6).
![]() |
(6) |
Step 6: MSN gateway receives the row coefficients from MSN sensor nodes. It re-organizes the set to get . It initiates the k- secure with FBKM process, using the 32x32 matrix is generated.
Step 7: Using the same procedure of step 4, an 8x8 matrix is generated and MSN gateway computes its row coefficients .
Step 8: MSN gateway verifies its coefficient with the coefficients sent by the MSN sensor node.
If the condition is satisfied, then MSN gateway authenticates the MSN sensor node as a valid node, otherwise, it rejects.
If then,
MSN sensor node is successfully authenticated by MSN gateway
else
MSN sensor node is rejected by MSN gateway
end
Step 9: MSN gateway forwards reaction to MSN sensor node specifying whether it is accepted or refused for the next level communication.
Step 10: MSN sensor node receives the authentication approval response from MSN gateway. Then, MSN sensor node encrypts the original data using the cypher key and generates the encrypted message.
Step 11: MSN sensor node then sends the encrypted message to MSN gateway.
Step 12: MSN gateway derived the decryption key similar to the encryption key by following Step 5 and decrypts the received message to get the original data.
Using this efficient key management technique, authentication is ensured between MSN sensor nodes and MSN Body Control Unit or gateway. Since this scheme is simple and robust, external or internal attackers cannot intrude into the network and manipulate patient data in any form. Since sensor nodes have limitations in memory, complexity, and power, this protocol is implemented efficiently. This protocol is designed for implementation between BSU and BCU. Therefore, this protocol can be used along with any key management technique, which will be implemented between BCU and destination point to improve the security of MSN.
4. RESULTS AND DISCUSSION
A typical simulated scenario of Medical Sensor Network to implement the authentication process is described in Fig. (3). X-axis and Y-axis in the network model plot refer to the coverage area 50 x 50 sq. km.
This platform consists of various medical sensors to measure the vital parameters of the patient. Medical sensors process the collected medical data and communicate them to MSN gateway; k-secure with FBKM scheme is implemented between sensor nodes and MSN gateway. Then, FBKM protocol is implemented between MSN gateway and MSN server.
In this scheme, the MSN gateway facilitates the execution of k-secure with FBKM algorithm as explained in the system model. This section presents the simulated results in respect with attacker and without attacker.
An attack is a kind of assault to obliterate, interpret, modify, immobilize, sneak or acquire unauthorized approach or make unauthorized utilization of an asset.
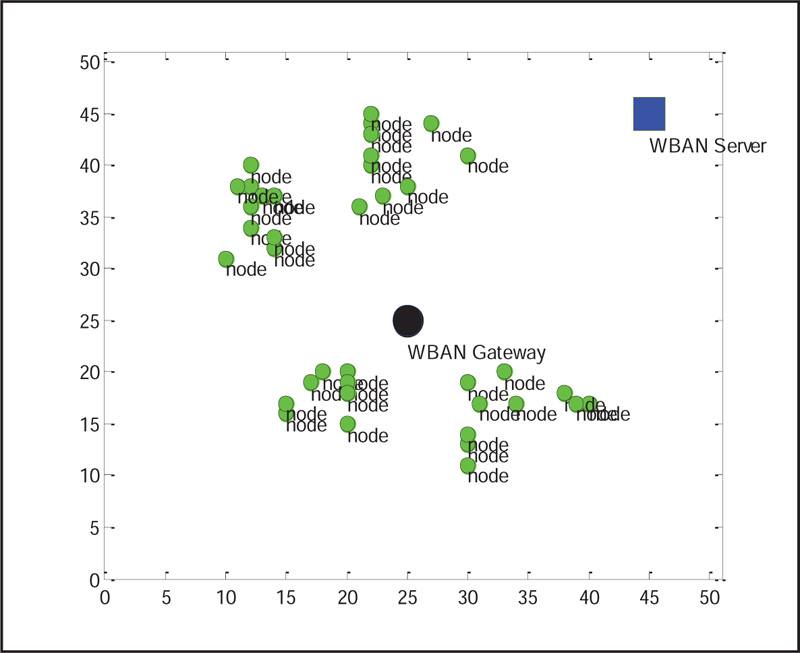
Without Attacker: According to the proposed algorithm, if the coefficients of MSN sensor nodes match with the coefficients of MSN gateway, then successfully, the connections are established between sensor nodes and gateway as shown in Fig. (4) under the assumption that the attacker is keeping quiet.
Now the connected sensor nodes can send the encrypted medical data to the gateway under successful authentication, as shown in Fig. (5).
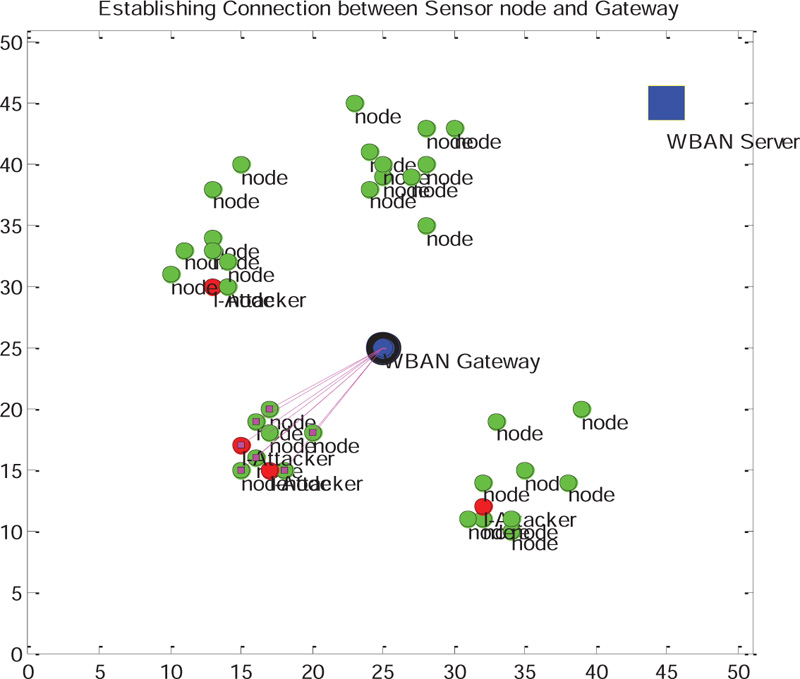
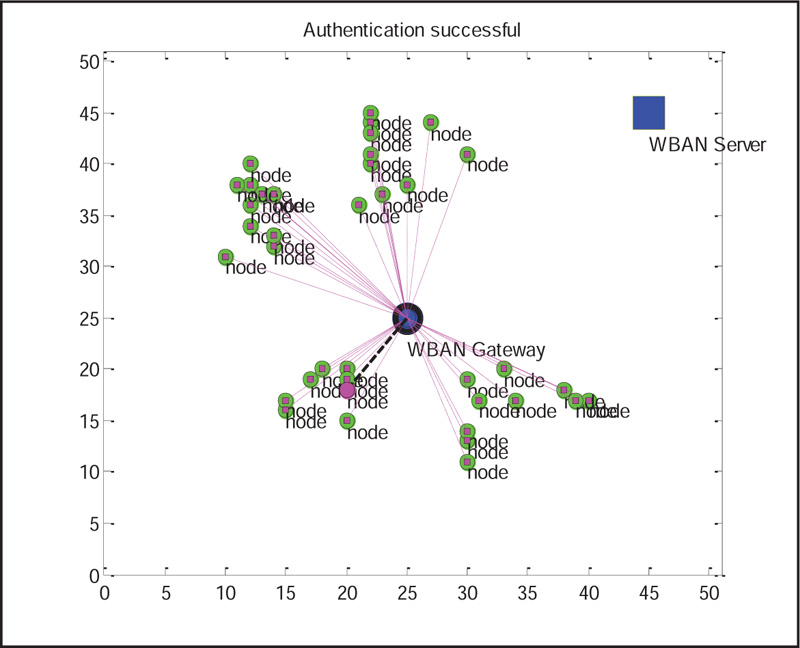
When the gateway is receiving the data, it can perform decryption to get back the original medical data. The successful authentication process is illustrated with sensor data and brain image as shown in Figs. (6 and 7).
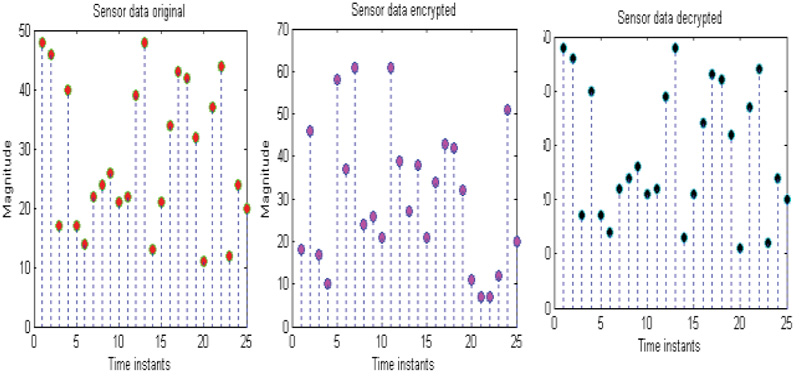
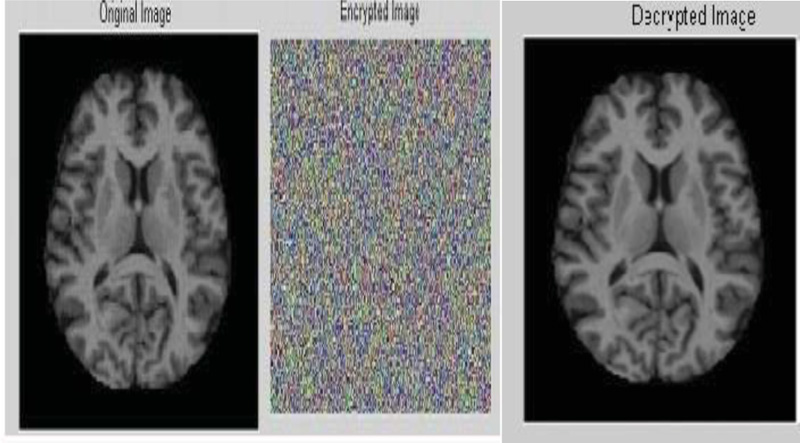
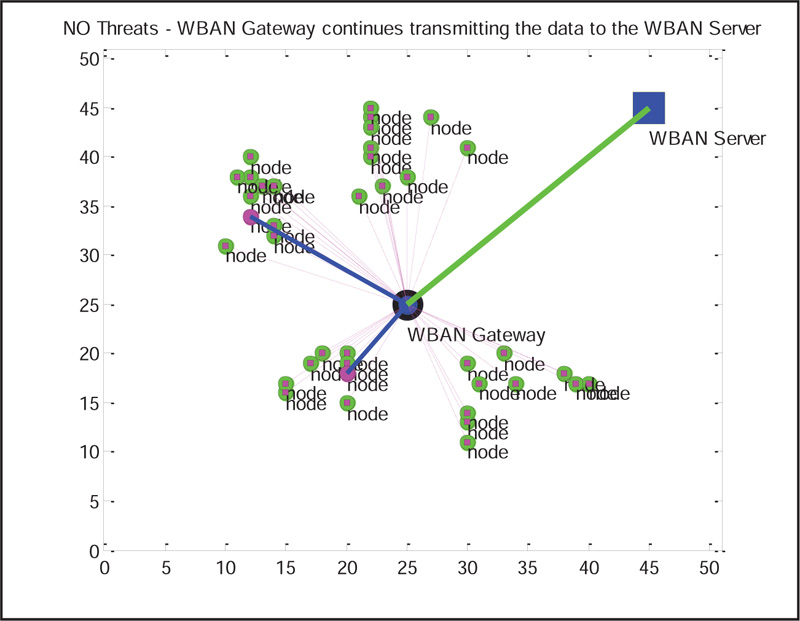
If the authentication is successful, the receiver can generate the cypher key, which will match with cypher key generated by the sender side. Therefore, it is true to accept the decrypted data or image as original data. From the gateway, it is suggested to apply FBKM algorithm, and data will be transmitted to the destination under the successful case, as shown in Fig. (8).
With Attacker: According to the proposed algorithm, if the coefficients of MSN sensor nodes do not match with the coefficients of MSN gateway, then the connections will not be established between sensor nodes and gateway. Then data communication will be blocked. This is because an unauthorized external attacker has tried to compromise the node to capture the patient data for manipulation. As k-secure with FBKM scheme is robust and unpredictable, it is not easy and possible for the attacker to construct the coefficients similar to the gateway; attacker fails to prove its authentication, as shown in Fig. (9).
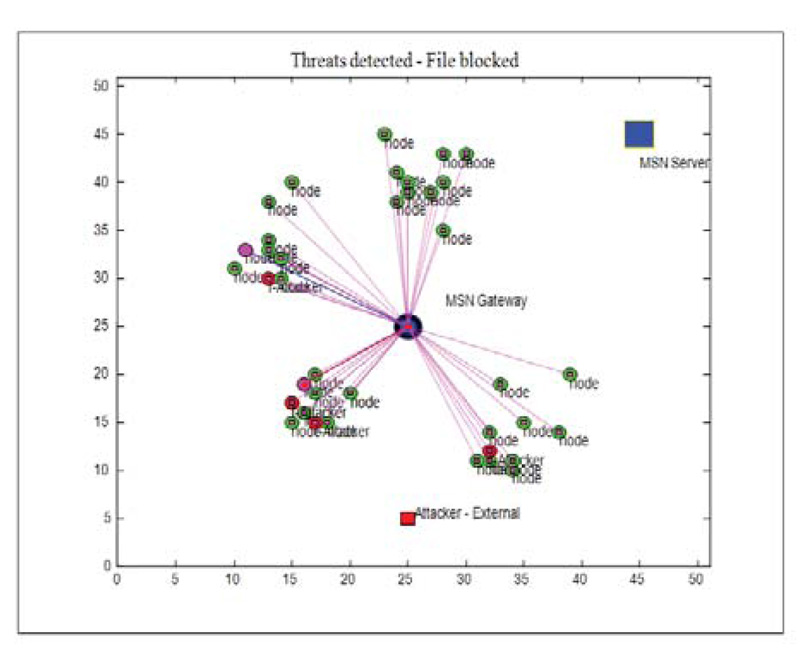
Fig. (10) shows the attempt made by the attacker to decrypt the data. But the attacker is not able to find the cypher key equivalent to cypher key generated by the gateway. Therefore, the attacker failed to decrypt the correct data as shown in Figs. (11 and 12).
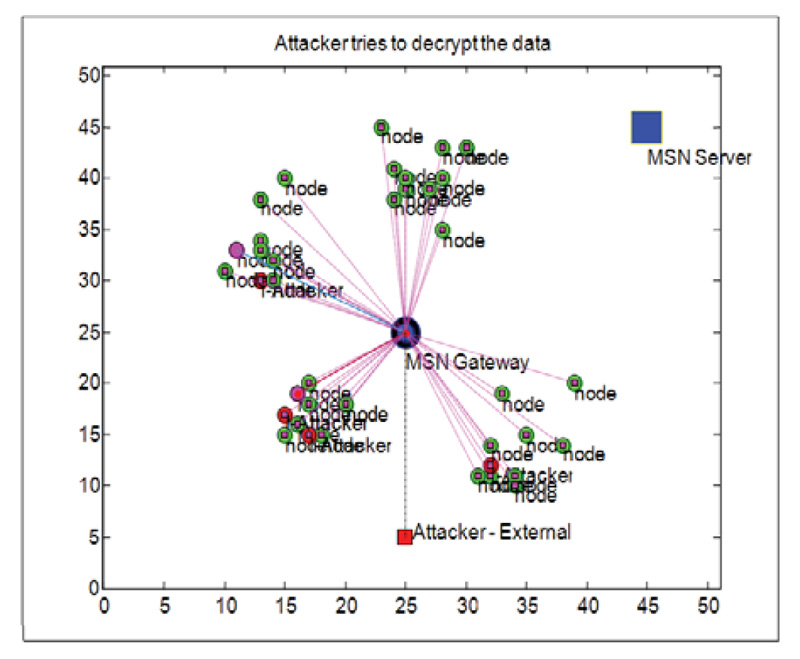
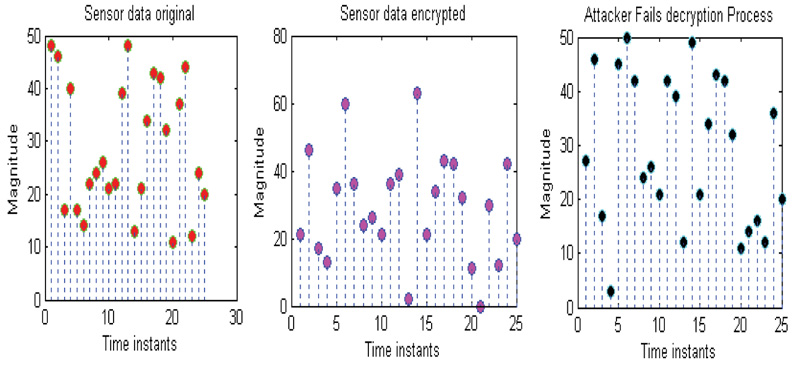
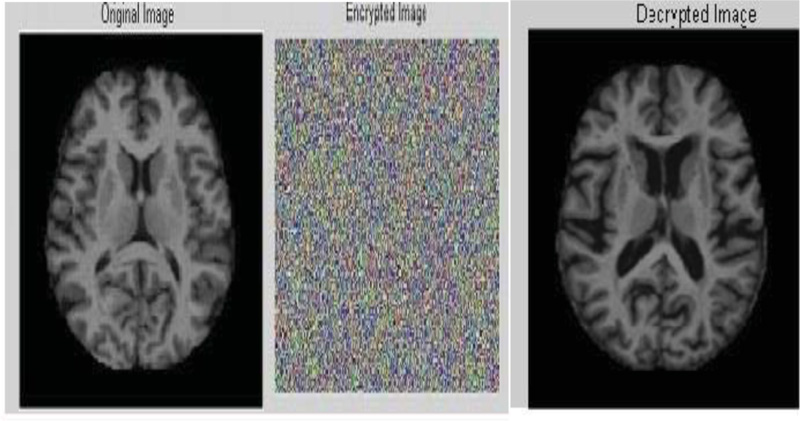
4.1. Receiver Operating Characteristic (ROC)
To analyse the performance of this algorithm, patient data has been taken from the MIT-BIH Arrhythmia Database. MIT-BIH Arrhythmia Database comprises 48 half-hour passages of two-channel ECG footages. They are digitized at 360 samples per second per channel with 11-bit resolution over a 10mV range. For explanation purpose, out of the 48 patient’s data, patient data 107.dat and patient data 203.dat have been taken into account. Performance of k-secure with FBKM scheme is evaluated by implementing this protocol along with FBKM scheme. It is reasonable to analyze ROC against FBKM scheme and ECG-IJS scheme. The Genuine Acceptance, in this case, is happening when an authorized person’s row coefficient formation and substantiation lead to a success when sensor nodes have the correct subset of the non-orthogonal matrix from gateway, including the success in FBKM scheme. A False Acceptance may occur when an unauthorized person’s effort for row coefficient formation and substantiations lead to success including the success in FBKM scheme.
The ROC curve drawn for the patient data 107.dat is shown in Fig. (13), which evidences that the crack built by authorized one’s success rate increases while the crack built by unauthorized attacker’s success rate is extremely minimal. Performance of k-secure with FBKM and FBKM algorithm produces better results than FBKM and ECG-IJS schemes. In k-secure with FBKM scheme, the combination of orthogonality factor for unique coefficient generation and the cypher key generation using unique pattern provides higher security for data transmitted between the sensors and the gateway. Here, when an eavesdropper hacks the information sent from a valid sensor to the gateway, the possibility for the attacker to identify the row coefficient for generating the unique pattern and the cypher key as per the algorithmic process of k-secure with FBKM scheme is very less. i.e., identifying the unique pattern and generating the correct cypher key within stipulated time by eavesdropper becomes cumbersome (without a similar unique row coefficient and a cypher key, the attacker would not be successful). This ensures data confidentiality as it prevents an attacker from injecting false data or modifying genuine data. Therefore, the GAR increases with lower FAR at the receiver end. Additionally, an extreme level of end-to-end security established between the body sensor unit and body control unit and between the body control unit and the destination end in k-secure with FBKM scheme ensures authenticity, confidentiality, and integrity at the MSN server increasing ROC compared to other existing schemes.
4.2. False Acceptance Rate (FAR)
False Acceptance Rate drawn for the patient data 203.dat is illustrated in Fig. (14). FAR Rate is described to bring out the incorrect verification of an unauthorized person. As per the procedure followed in FBKM scheme, FAR is calculated and it is shown that FAR decreases for the polynomial degree ‘D’ between 5 and 10 for the tolerance value 2. From Fig. (14), it is obvious that k-secure and FBKM provide an excellent result when compared with FBKM alone and ECG-IJS. This is because the proposed k-secure with FBKM algorithm implemented in the local unit assures correct data access(originated from a valid source) at the gateway and finally at the server. The end-to-end secured data transmission of the proposed scheme facilitates low FAR at the server compared to other schemes.
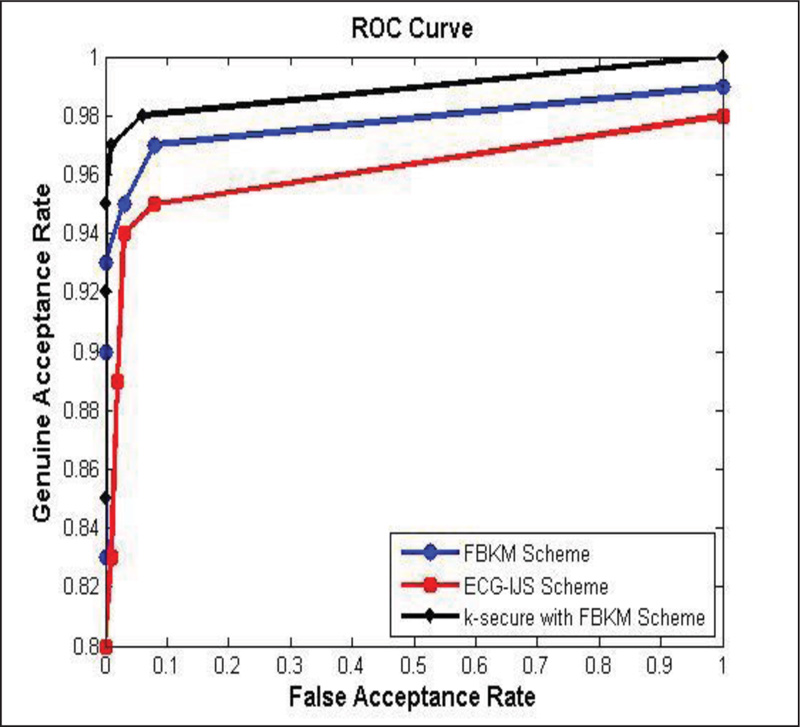
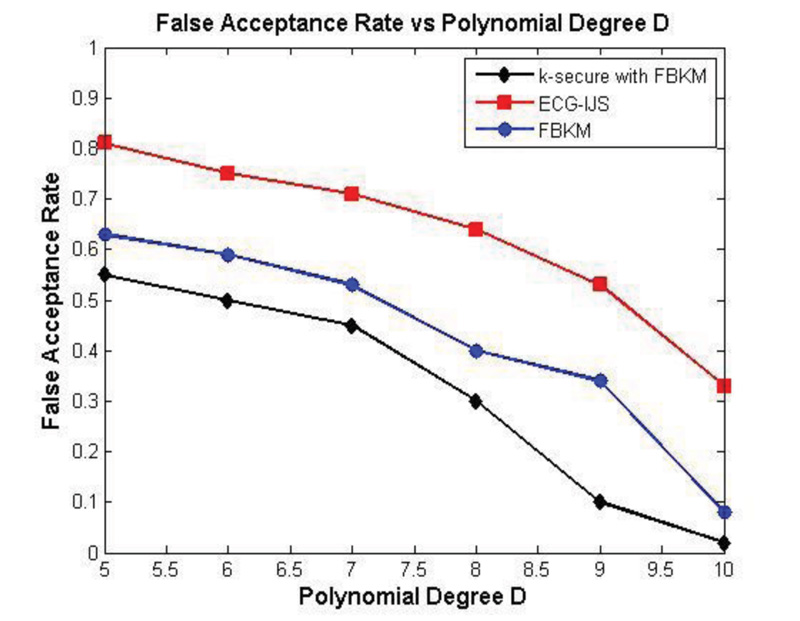
If an attacker tries to eavesdrop on the information sent from the sensor to the server, the attacker would fail in generating the original message due to the superior level of security provided by the k-secure with FBKM scheme. Here, a combination of unique row coefficient and cypher key generation facilitates secure data communication between the sensor and the gateway while security validation through defuzzification and vault key unlock ensures the security aspects, namely, concealment and veracity for information transmitted between the gateway and the server. These security measures reduce FAR even when D is low and t is increased in the proposed scheme compared to others.
4.3. False Rejection Rate (FRR)
FRR performance for the patient data 203.dat is shown in Fig. (15). The FRR is described to bring out the incorrect rejection of an access attempt by an authorized person. From Fig. (15), it is understood that FRR increases when the polynomial degree ‘D’ increases. K-secure with FBKM provides outstanding result when compared with FBKM and ECG-IJS for the tolerance value 2. This is because the proposed k-secure with FBKM algorithm employed in the local unit identifies an authorized person at gateway itself. This improves the complete picture of the MSN scenario. The unique row coefficient and cypher key generation facilitate acceptable FRR at the gateway. As the malicious node attempts to modify the packet transmitted between the sensor and the gateway, it fails due to the security incorporated during data communication.
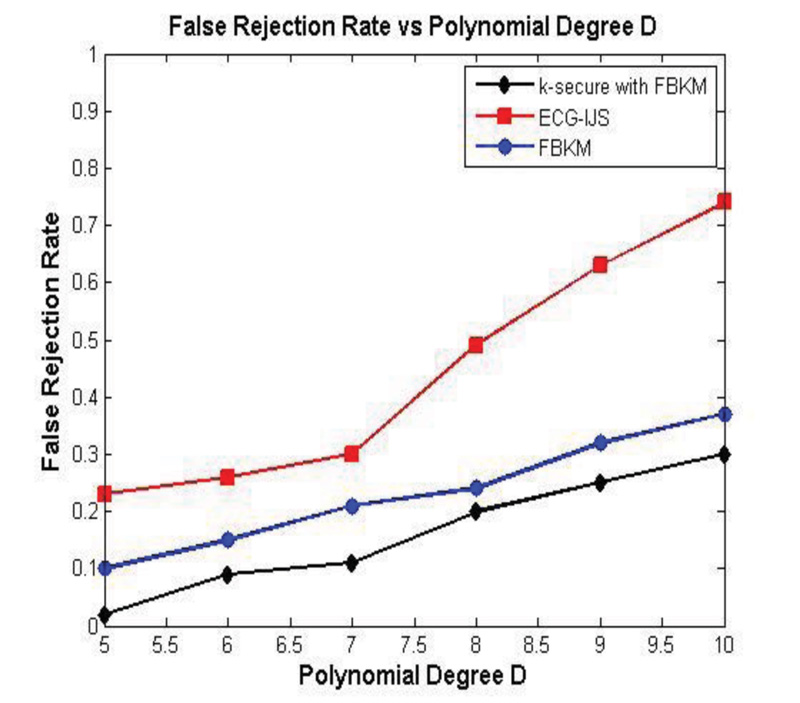
The proposed scheme makes the system reliable by protecting the confidentiality, integrity, and availability of network data from malicious attacks, which can lead to information disclosure. Unlike other schemes, this scheme is a computation-intensive process; the proposed model incorporates an effective authentication mechanism that proves to be highly secured on sensor devices, gateway, and the server. Once the authentication by the self-assured body control unit is successful for the body sensor units, it becomes an accredited and a faithful object to transfer data to the gateway. Additional security measures implemented by the gateway as per the FBKM scheme functionality assures false data injection or message modification, thereby ensuring correct acceptance of information originated from an authorized source and prevents incorrect rejection of information from the correct source. Thus, the comparison analysis of FRR indicates that k-secure with FBKM scheme’s incorrect rejection for authorized users when compared to other existing schemes.
4.4. Genuine Acceptance Rate (GAR):
Genuine Acceptance Rate performance for the patient data 203.dat is shown in Fig. (16). The Genuine Acceptance, in this case, is enhanced due to the execution of an authentication process between sensor nodes and MSN gateway. The proposed k-secure with FBKM algorithm assures the originality of the data and the authorized user at the MSN gateway point. As per the procedure followed in FBKM scheme, it is understood that GAR decreases when the polynomial degree ‘D’ increases for the tolerance value 2. The comparison results indicate that the Genuine Acceptance Rate of k-secure with FBKM is higher than ECG-IJS scheme and FBKM scheme.
4.5. Half Total Error Rate (HTER)
The HTER is the mean of FAR and FRR. Half Total Error Rate performance for the patient data 203.dat is illustrated in Fig. (17); when the polynomial degree ‘D’increases, HTER decreases for the tolerance value set to 2. The comparison results indicate that the Half Total Error Rate of k-secure with FBKM is less than ECG-IJS scheme and FBKM scheme. Thus k-secure with FBKM scheme is found to be an extremely robust scheme with minimum error.
The main advantage of this algorithm is that it is less complex to satisfy the constraints of medical sensors memory requirement, size and energy requirement. K-secure with FBKM algorithm is designed to counteract both internal and external attackers. The keys generated by this algorithm are random and distinct. The combination of k-secure with FBKM and FBKM algorithm implementation from end to end assures the originality of the data and satisfies all the security aspects. This combination of algorithms reveals good performance by providing low FRR, low FAR, high GAR, and low HTER.
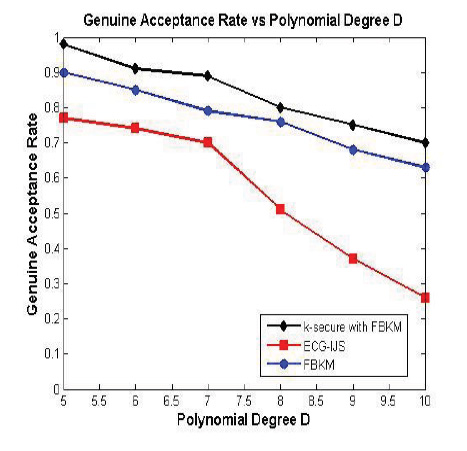
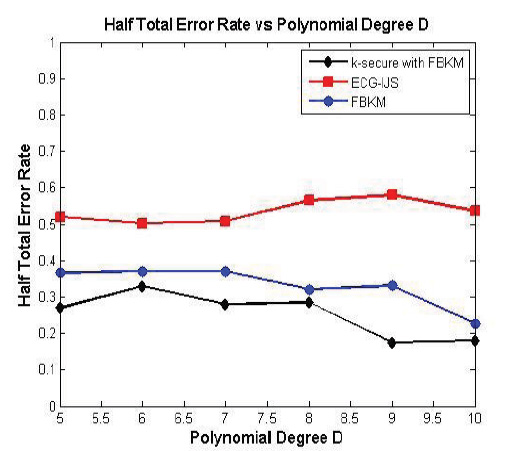
CONCLUSION
It is mandatory to have a protected platform that ensures security aspects, namely, authentication, non-repudiation, confidentiality and integrity for sending and receiving sensitive medical information in MSN to assure life expectancy. Particularly in various situations, attackers and intruders are a threat to people. This article has proposed a robust key management technique k-secure with FBKM scheme to be applied between body sensor units and body control unit. The experimental results discussed in this article show that the results of the existing FBKM scheme in Medical Sensor Networks are improved for better performance in telemedicine applications. In the future, it is planned to improve the security level of the k-secure with FBKM scheme by increasing the non-orthogonal matrix size, implementing the scheme on hardware, and investigating the implementation of the proposed scheme for economical memory and power requirements.
ETHICS APPROVAL AND CONSENT TO PARTICIPATE
Not applicable.
HUMAN AND ANIMAL RIGHTS
Not applicable.
CONSENT FOR PUBLICATION
Not applicable.
AVAILABILITY OF DATA AND MATERIALS
The data sets used during the current study can be provided from the corresponding author (K.K) ,upon reasonable request.
FUNDING
None.
CONFLICT OF INTEREST
The authors declare no conflict of interest, financial or otherwise.
ACKNOWLEDGEMENTS
Declared none.