All published articles of this journal are available on ScienceDirect.
Brain-Computer Interface for Persons with Motor Disabilities - A Review
Abstract
Aim /Objective:
A Brain-Computer Interface (BCI) is a communication medium, which restructures brain signals into respective commands for an external device.
Methodology:
A BCI allows its target users like persons with motor disabilities to act on their environment using brain signals without using peripheral nerves or muscles. In this review article, we have presented a view on different BCIs for humans with motor disabilities.
Results & Conclusion:
From the study, it is clear that the P300 based Electroencephalography (EEG)BCIs with Steady-State Visually Evoked Potential (SSVEP) non-parametric feature extraction techniques work with high efficiency in the major parameters like Information Bit Transfer Rate (ITR), Mutual Information (MI) rate and Low Signal to Noise Ratio (SNR) and achieve a maximum classification accuracy using Self Organized Fuzzy Neural Network (SOFNN).
1. INTRODUCTION
The human brain has a huge network of nervous cells/neurons. The neurons fire inside the brain and produce an electrical signal. These neurons transmit information from the brain to discretionary muscles to control the motor organs [1]. When the neurons are not able to transmit signals among the brain and nervous system, these disorders lead to coma and paralysis. Such kind of individuals needs Brain-Computer Interface (BCI) for communication [2]. BCI systems enable a person with motor disabilities to send commands to a device by means of brain signals [3,4]. In order to control a BCI, the user must have to produce brain activity patterns that can be transmitted to the system and translated into commands [5].
The BCI system (Fig. 1) has subsequent components: (i) a tool to record brain functionaries either invasive Electrocorticography (ECoG) [6] or non–invasive Electroencephalography (EEG) [7]; (ii) a preprocessor that minimizes the artifacts and noises [8]; (iii) a decoder which decodes the preprocessed signal into an impact signal [9] for (iv) an adscititious tool that might be a suitable application for the BCI (e.g., an automatic mechanism, a display monitor etc.) [10] [11]. The opinion of the user was a vital feature of the BCI model because it gives the previous input concerning errors. With this, it encourages the user to regulate the activity of the brain to extend consideration and involvement within the work or a task, therefore, observing to a neurofeedback principle, the BCI is often considered as an impactful model with the active response (closed-loop system) [12-15]. A BCI could be a communication system that is not based on the brain’s conventional fasciculus output channels. The user’s objective is delivered through brain signals (EEG) instead of by peripheral muscles and nerves, and these signals of the brain do not rely on his or her production on fasciculus actions. The BCI provides a period of communication to the user and also to the external world. The user gets a reflective response as the result of the BCI tasks, which response will have an effect on the user’s resultant objective and its expression in signals from the brain.
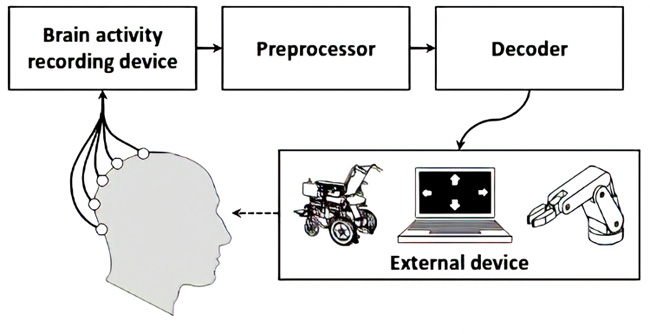
2. BRAIN-COMPUTER INTERFACE TYPES
There are many forms of BCI determining the essential reason for those tools to block the electrical signals which pass among neurons within the brain and convert it to a signal which is detected by external tools.
2.1. Invasive BCI
Invasive BCI tools are those deep-routed directly into the brain to acquire signals without artifacts. Invasive BCIs do not work with ECoG signals, hence they utilize direct brain signals to operate leg, limbs, and arms. As they rest inside the grey substance, invasive tools make the best signals of BCI devices; in any case, they are at the risk of scaring brain -tissues, perpetrating the signal to turn out vulnerable or maybe lost that the body responds to an external object within the brain.
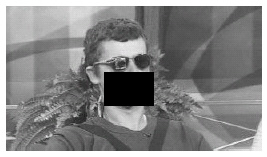
BCIs specializing in motor neuroprosthetics mean to either reestablishing development in paralytic individuals or to provide devices for supporting them, like interacting with computers or robotic arms. This experiment utilizes a lot of refined embedded based active mapping of phosphenes as appeared in Fig. (2).
2.2. Partially Invasive Brain-Computer Interfaces
Partially invasive BCI systems are deep-routed inside the skull, and rest is fixed externally from the brain, rather than inside the grey substance. Signal quality exploitation, this sort of BCI is somewhat vulnerable once it correlates to Invasive BCI. They generate high-quality signals than non-invasive BCIs. Partly invasive BCIs have the minimum threat of connective tissue development when placed beside Invasive BCI.
2.3. Non-Invasive BCI
The non-invasive method is one within which the clinical scanning sensors or devices that are fixed on caps or headbands to scan the brain signals. Here, electrodes cannot be fixed straightforwardly on the predefined region of the brain but on the scalp. One of the very popular devices beneath this class is that the EEG equipped device to present fine temporal resolutions. It is easy to utilize, minimal cost and transportable. Non-invasive BCI has the lesser signal clarity once it includes human action with the brain (skull distortion signal) which is most secure of all sort. This type of device has been utilized to make progress in enabling a patient to move muscle implants and reestablish partial movement.
ECoG is a non-invasive technique. Here, the electrodes are implanted in a thin plastic pad which is fixed on the cortex, at a minimum place with respect to the meninx. ECoG based robotic BCI devices utilize one-dimensional control utilization. Light-weight Reactive Imaging BCI devices are still inside the realm of theory. These would include embedding optical tool inside the bone. The optical tool would be trained on a single nerve cell. In this manner, each neuron's coefficient will be estimated by a single detector. When nerve cell fires, the optical tool’s light-weight pattern and its wavelength reflection would modify marginally so as to reduce the chance of scar tissue development through non-invasive ECoG.
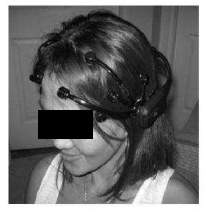
2.4. The Emotiv Education Edition SDK
The EEG headset receiver, to extricate an individual's brain waves to diagnose them, is an EPOC headset receiver. Insights of an EPOC headset receiver are defined below. The Education Edition SDK by Emotiv Systems has a headset: a fourteen channel (in addition to CMS/DRL references, P3/P4 regions) high resolution, neuro-signal obtaining and process wireless neuro-headset receiver as represented in Fig.(3) &. (4) Channel names upheld the International 10-20 regions are: AF3, F3, F7, FC5, P7, T7, P8, O1, O2, T8, F4, FC6, F8, &AF4 [16].
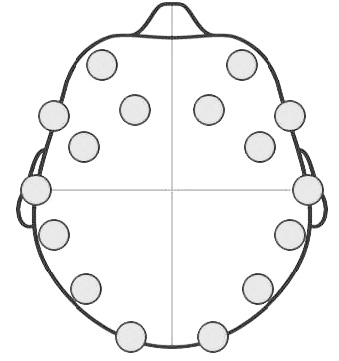
The Education Edition SDK moreover comprises a restrictive package toolbox that uncovers the Apis and identification libraries. The SDK gives a better improvement setting that combines well with new and existing systems. Various methods for obtaining brain signals epitomize EEG and Magnetoencephalography (MEG). These methods are being considered of MRI and Near-Infrared Spectroscopy (NIRS) to provide an analysis of brain wave and chemical patterns; in any case, they are impractically inferable due to their size [17].
2.5. P300 EEG Based BCI
The P300 is an Event Relatedpotential, a quantifiable electrical charge that is linearly associated with impulse. A P300 BCI will linearly make an interpretation of an individual's intention into electrical signals which control artificial devices [18,19]. A P300 speller is predicated on this standard, the identification of P300 waves allows the user to enter characters. The P300 speller comprises of two various classification issues. The main classification is to locate the existence of a P300 inside the graphical record (EEG). The second relates to the combination of various P300 reactions for a significant possible character to spell [20].
3. FEATURE EXTRACTION
In a BCI framework, the extraction of the feature begins from an underlying set of estimated data and develops determined qualities (features) intended to be instructive and non-repetitive, facilitating the next learning and speculation steps. The extraction of features is said to be spatiality reduction. When the input file to an algorithmic program is simply too large to process and it is assumed to be excess, at that point, it will be renovated into a reduced set of choices. This technique is named feature selection. The selected option squares the measure of predicting important data from the input file, so the predefined task will be performed by mishandling this reduced outline instead of the entire initial data.
The extraction of the feature includes lessening the number of resources required to clarify a large set of data. When performing analysis of different data, one among the first issues originates from the number of factors considered. Analyzing with a larger usual range of factors needs a large amount of memory and computation power; collectively, it should cause a classification algorithmic program to overfit to instruct the samples and sum up ineffectively. The extraction of features could be a common term for developing combinations of variables, to initiate the input with adequate accuracy. From the study [21-29], several high-performance feature extraction techniques have been reported, as listed in the summary. The majority of the techniques listed from the study [21-29] are reported with non-parametric types of feature extraction techniques, the study [29] alone used parametric AR method for feature extraction.
4. CLASSIFICATION ALGORITHMS OF BCIS
The classification step followed throughout the BCI system is to acknowledge the subject’s activity with the support of a feature vector and to classify it. Either regression or classification algorithms are utilized [30]. The classification algorithms use alternatives of extricated variables for stipulating boundaries among the feature sets [31]. As represented the difference among any two modifications, for a two-target case, each of a regression method or a classification method is desired to have the parameter of 1 that may be operated throughout the distributed area directly. In distinction, the classification method works with the perseverance of 3 functions, one for every 3 boundaries among the 4 targets.
Therefore, the classification method may collectively be helpful for two-target applications and additionally, the regression method is utilized for larger targets, once these targets are organized on one or many dimensions. Additionally, the regression method was efficient for constant feedback i.e. application that comprises constant control of movements [32]. In comparison, an on-line session gives away BCI framework analysis throughout real-time conditions. This means, offline simulation and cross-validation are effective ways to develop and correlate new algorithms.However, separate on-line analysis will result in better BCI performance [33-46]. The classification algorithm has been labeled by users through supervised learning in the feature extracted dataset.
5. DISCUSSION
The majority of the individuals with disabilities will have to manage their activities using brain signals, the EEG signals were mostly an applicable choice of input signals for the BCI system. EEG signals were acquired from channels associated with persons with motor disabilities. The corresponding commands were derived from EEG signals, which support the brain signal pattern. If the amplitude recorded from the brain signals throughout the trials was larger than the limit, then the system identifies a command for processing. For e.g. “turn right” and “turn left” command is detected, whereas positive or a negative potential recorded from the brain signal seems larger than the connected threshold. If each value were matched with the threshold value, there will be “no action”, brain signal order was identified. The common benchmarked electrode placements are C21, C23, A1, A3, A19, A20, and A21, which are mostly used in BCI experimentations. As far as the conventional P300 were concerned, they deal with Event-Related Potential (ERP) related stimulus, such type of stimulus produces comparatively less accuracy than the SSVEP based visual stimulus techniques. The SSVEP is an oscillatory signal that evokes in the occipital lobe of the EEG in reaction to a visual stimulus, which was modified at a certain frequency. In SSVEP based BCIs, the visual stimulus was modulated at various frequencies. Once a BCI user aims attention on a bound pattern, the related stimulating frequency predominantly generates in the spectral illustration of the EEG signal obtained at occipital sites, such action connected to the dominant frequency were executed [47,48]. BCI study [27] presents a high-speed speller BCI with a rate of conveying 40 characters that have been presented for 13 subjects involving in visual stimulus, hence this study proves that SSVEP has been a promising analysis. In feature extraction methods, SSVEP based non-parametric extraction technique has given more information like time-domain features and spatiotemporal features, which were most predominant than the ERP based Parametric feature Extraction Technique. Many neural network classifiers like SVM, PNN were presented in several BCI studies but the classification accuracy seems comparatively lesser than the hybrid variety of neural network classifiers (e.g., (SOFNN)). In SSVEP based visual stimulus,SOFNN Classifiers are more predominant in processing the raw EEG signals such that it spontaneously evokes Mu and Beta frequencies for Right and left-hand motor imagery movements and achieves classification accuracy with high ITRs and low SNR [49-60]. Several analyses had the accuracy of BCIs and produced suitable data bit rate, though the inherent major challenges in the brain signal process. However, the recent vital improvements, issues, and problems are yet to be resolved. Such tasks were performed using invasive, non-invasive and partially invasive BCIs for the persons with motor disabilities. Majority of the researchers ignored partially invasive and invasive methodology due to its practical difficulties. In common, speller based BCIs with the combination of Brain signal to audio and brain signal to text with SSVEP parametric feature extraction seems to be much efficient than the conventional type of BCIs. For resolving the practical drawbacks, a new concept of P300 with Global System for Mobile Communications (GSM) based BCIs with SSVEP based Statistical feature extraction technique for EEG operations with an additional operation of transmitting the brain signals to text as well as audio during emergency will perform more efficiently than the above existing conventional type of BCIs for reducing the practical difficulties of a person with motor disabilities.
CONCLUSION
This work has analyzed the progression of BCI systems, discussing essential parts of different BCI models and the most significant objectives that have driven BCI analysis in the past twenty years. It has been noticed that a lot of progress has been made in the analysis of BCI. Various neuroimaging methodologies are included in this progress which are to be implemented in BCI; (I) EEG, that presents satisfactory quality signals with high versatility and is beyond the regular methodologies; (ii) fMRI works with large integer scale, that squares the measure of attempted viable methods for confining active zones inside the brain; and (iii) Invasive methods, that are significant techniques to deliver high quality signal that are required in some dimensional control applications e.g. neuroprostheses management. BCI studies have considered time, frequency, and spatial components of brain signals individually. These signal dimension interdependencies are the latest developments in BCI. BCI analysis suggests that creative improvements are awaiting in the near future. These accomplishments and furthermore the potential for brand new BCI applications have clearly given a valuable improvement to BCI analysis including multidisciplinary researchers e.g. neuroscientists, mathematicians, engineers, and medical rehabilitation experts, among others. Interest within the BCI domain is predicted to expand based on the possibilities. In the future, the BCI system may become another model of human-machine combination with various levels in the day to day life of individuals with motor disabilities.
Authors (Year) |
Sample size / Subjects& Stimulus Duration |
Stimuli Frequency Range (Hz) |
Stimulus Levels |
Analysis Method/ Feature Extraction |
Reports/Results |
---|---|---|---|---|---|
Pieter-Jan Kindermans, et.al, (2014) |
10 Samples & 125 ms | 256Hz | 300ms, post stimulus | ERP Features | High efficient Unsupervised P300 speller BCI has been presented |
Dandan Huang, (2012) |
5 Healthy Subjects (2 out 5 Subjects got 200 samples) |
256Hz | Not Applicable | Spatio-Temporal Features | High-Performance 2D BCI wheelchair has been Reported, with an average classification accuracy of 70% to 80% |
Eric C Leuthardt, et.al, (2006) |
4 subjects 3male & 1 female |
180Hz | 12-time intervals, post-stimulus | ECoG Features (Amplitude in specific Frequency bands) | ECoG based BCI scheme is more efficient than EEG based BCIs. All the four controls achieved 73% to 100% of performance efficiency, but this method is invasive. |
Damien Coyle, et.al, (2005) |
3 healthy Subjects | 128Hz | Not Applicable | Self-Organizing Fuzzy Neural Network-based Time Series Prediction (Statistical Time Features) |
High Efficient classification accuracy, Information transfer rate (ITR) and Mutual Information (MI) rate have been achieved. |
YaninaAtum, et.al, (2010) |
Single Subject | 1024Hz | Not Applicable | Discrete Dictionary-based Feature Extraction Approach | The wrapped wavelet samples represent the best performance over the temporal patterns. |
Pieter-Jan Kindermans, et.al, (2012) |
Akimpech P300 database, which covers 22 subjects performing Spanish Language Spelling | 256Hz | 300ms, post-stimulus | ERP Features | Results show Unsupervised P300 speller models perform better than supervised models in specific areas. |
Xiaogang Chen, et.al, (2012) |
12 subjects | Not mentioned | Stimulation Levels varies between each subject from (o – 1s) | SSVEP Features | High performance, High speedSpellerBCI for communication has been reported, with high-speed information transfer rate of 5.32 bps |
Masaki Nakanishi, et.al, (In Press) |
13 Subjects | Not mentioned | Visual Stimulus is used, Stimulus levels Not seen |
SSVEP (time-domain) Features | High-Speed SSVEP- BCI in real timeapplications. Achieved an average of 166.91 bits/min for Information Transfer Rate. |
C. Guger, et.al, (2009) |
100 subjects 32 female and 68 male |
256Hz | 800ms, Post Stimulus | Features are not apparently seen but LDA is used to choose the accurate features. | High accuracy has been reported for the persons with motor disabilities, with a spelling accuracy of 80% to 100% |
Tie-Jun Liu, et.al, (2009) |
3 subjects | 1000Hz | Not Applicable | AR feature extraction Model | Real-Time BCI- System Based on Motor Imagery is reported with the best accuracy levels. |
CONSENT FOR PUBLICATION
Not applicable.
AVAILABILITY OF DATA AND MATERIALS
Not applicable.
FUNDING
None.
CONFLICT OF INTEREST
The authors declare no conflict of interest, financial or otherwise.
ACKNOWLEDGEMENTS
Declared none.